Sora is an AI video synthesis model trained on a large scale of video data, which can create realistic and imaginative scenes according to text instructions. OpenAI is teaching artificial intelligence to understand and simulate the physical world in motion, with the goal of training models to help people solve problems that require interaction with the real world. Currently, Sora can generate videos up to one-minute-long on user input, while reasonably arranging the physical relationships between multiple people in the video, with high-definition image quality.
Awesome Video Synthesis
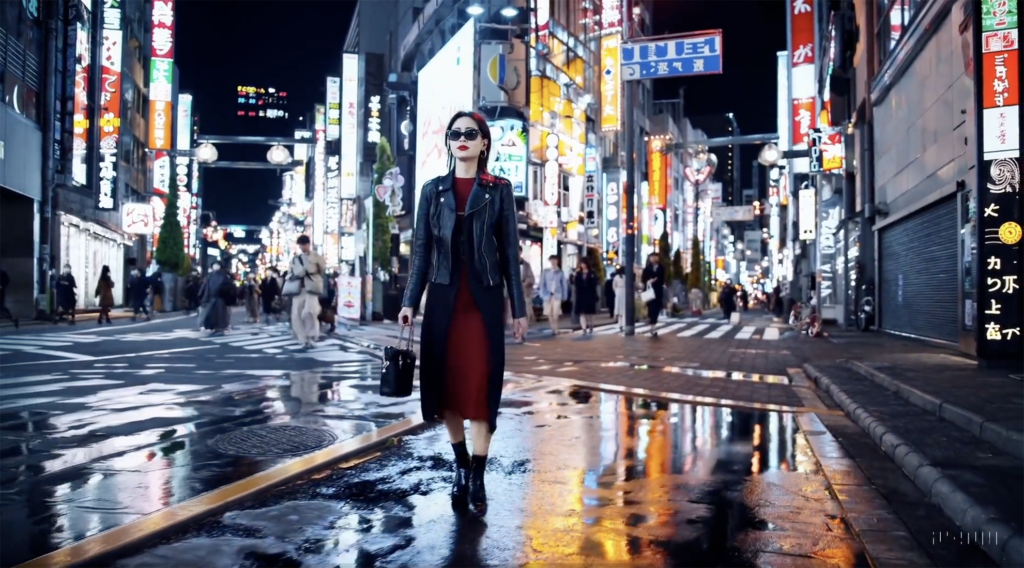
A stylish woman walks down a Tokyo street filled with warm glowing neon and animated city signage. She wears a black leather jacket, a long red dress, and black boots, and carries a black purse. She wears sunglasses and red lipstick. She walks confidently and casually. The street is damp and reflective, creating a mirror effect of the colorful lights. Many pedestrians walk about.
Technical Report of Sora
The recent technical report from OpenAI on Sora failed to disclose specific details about the model and its implementation. The report only provides an introduction to data processing and quantitative evaluation. Prior work on video synthesis before Sora has employed various methods to study the generation modeling of video data, including recurrent networks, generative adversarial networks, autoregressive Transformers, and diffusion models. These efforts typically focus on specific categories of visual data, shorter videos, or fixed-size videos. In contrast, Sora is a universal visual data model capable of generating videos and images of varying lengths, aspect ratios, and resolutions, with the longest being a high-definition video of up to one minute.
Turning Visual Data Into Patch
OpenAI, inspired by Large Language Models (LLMs), which acquire general capabilities by training on internet-scale data, considers how the paradigm’s success partly owes to the use of tokens, which cleverly unify various forms of text—code, mathematics, and various natural languages. In this work, OpenAI investigates how generative visual data models can inherit these advantages. Unlike LLMs with text tokens, Sora employs visual patch. It has been demonstrated previously that visual patch are effective representations for visual data models. OpenAI finds that visual patch are highly scalable and effective representations, suitable for training generative models on various types of videos and images.
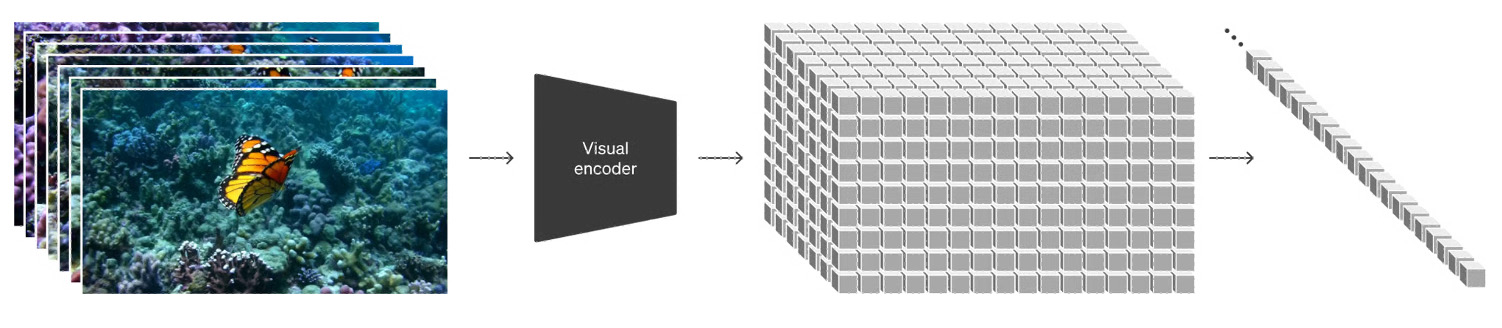
In high-level terms, the process involves first compressing the video into a lower-dimensional latent space and then decomposing the representation into spatiotemporal blocks (patch), transforming the video into blocks (patch).
Video Compression Network
OpenAI trained a network that can reduce the dimensions of visual data. This network takes raw videos as input and outputs a compressed latent representation in both time and space. Sora is trained on this compressed latent space and subsequently generates videos. OpenAI also trained a corresponding decoder model to map the generated latent representation back to pixel space.
Spacetime Latent Patch
Given a compressed input video, OpenAI extracts a series of temporal-spatial patches, which act as Transformer tokens. This approach is also applicable to images, as images are just videos with a single . OpenAI’s patch- d representation allows Sora to be trained on videos and images with variable resolutions, durations, and aspect ratios. During inference, OpenAI can control the size of the generated video by arranging randomly initialized patches in appropriately sized grids.
Scaling Transformers for Video Generation
Sora is a diffusion model; given noisy input patches (along with conditional information like text s), it is trained to predict the original “clean” patch. Crucially, Sora is an extended Transformer. Transformers have demonstrated excellent scalability in various domains, including language modeling, computer vision, and image generation. In this work, OpenAI found that extended Transformers can effectively scale in video models as well. OpenAI observed improvements in sample quality with increasing training compute for video samples with fixed seeds and inputs. As training compute increases, sample quality significantly improves.
Variable Duration, Resolution, Aspect Ratio, Sampling Flexibility
Sora demonstrates robust support for diverse video formats. For instance, whether it’s widescreen 1920x1080p videos, vertical 1080×1920 videos, or videos with other arbitrary ratios, Sora can handle them seamlessly. This capability allows Sora to directly generate content in the native aspect ratio for different devices, catering to varying viewing needs. Additionally, Sora can quickly prototype content at lower resolutions and then generate it at full resolution, all within the same model. This feature not only enhances the flexibility of content creation but also greatly simplifies the video content generation process.
Improved Composition and Framing
Furthermore, Sora shows significant improvements in video composition and framing. By training on native aspect ratios, Sora can better grasp the composition and framing of videos. Compared to models that crop all training videos into squares, Sora can more accurately preserve the overall theme of the video. For example, for widescreen format videos, Sora can ensure that the main content remains in the viewer’s line of sight, unlike some models that only show a portion of the subject. This not only enhances the visual quality of generated videos but also improves the viewing experience.
Natural Language Understanding
Training a text-to-video generation system like Sora requires a large number of videos with corresponding textual descriptions. OpenAI applies the re-captioning technique introduced in DALL·E 3 to videos. OpenAI first trains a highly descriptive captioning model and then uses it to generate text captions for all videos in OpenAI’s training set. OpenAI found that training on highly descriptive video titles can improve the fidelity of the text and the overall quality of the videos. Similar to DALL·E 3, OpenAI also utilizes GPT to transform users’ shorts into more detailed long titles, which are then fed into the video model. This allows Sora to generate high-quality videos accurately following users.
Sora’s Training Data
The data used behind Sora has also become a hot topic in the AI industry. Just recently the OpenAI Sora team mentioned data related content in an interview:
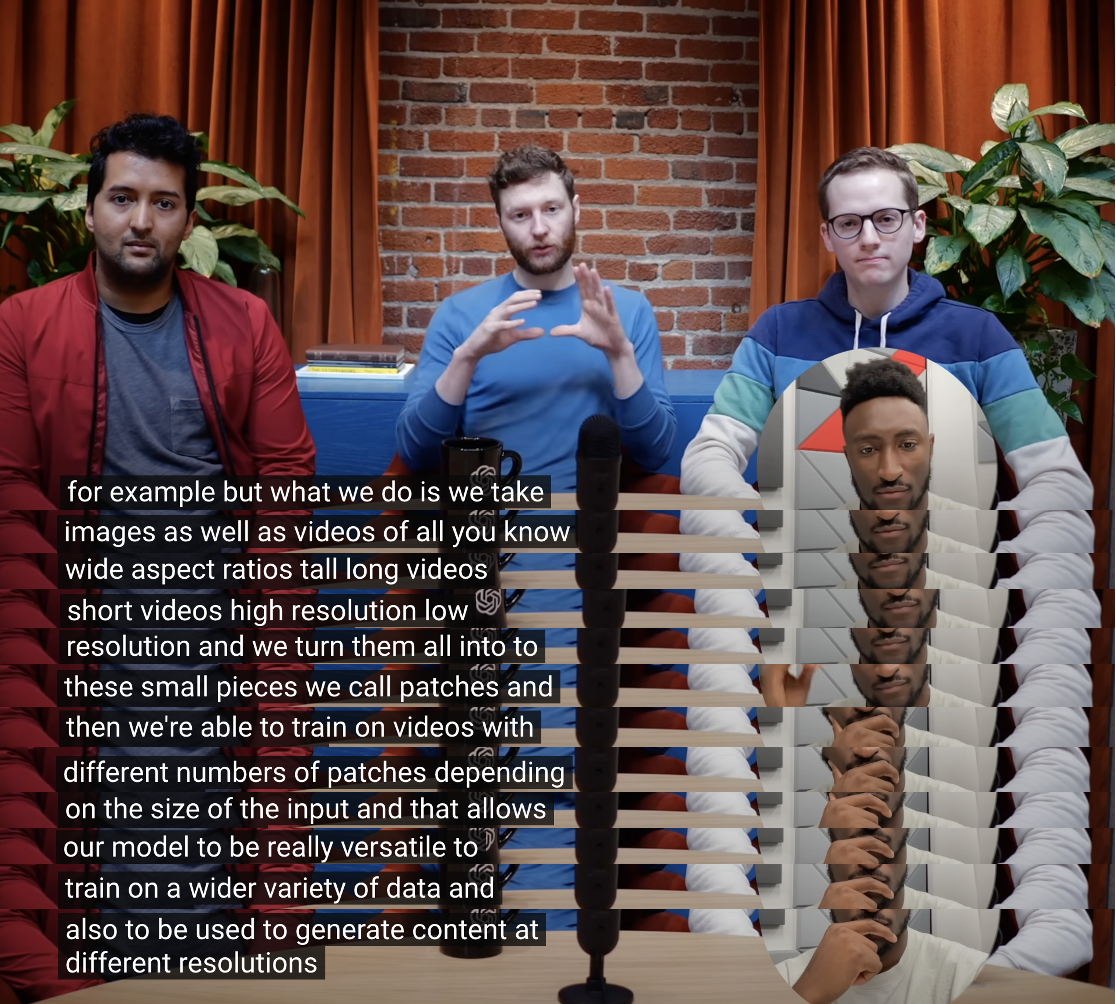
Although model-generated data can enhance the performance of the model to a certain extent, it can never be comparable to the accuracy and robustness of real data. DataoceanAI always focuses on providing high-quality data services and resources.
Since last year, we have joined hands with leading LLMs companies to co-create the data solutions for multimodal large language model, and we are committed to creating an all-round one-stop data service experience for customer. Our services cover data acquisition, preprocessing, cleaning, labeling and quality inspection to ensure that customers can get the best quality datasets.
Video Caption Data Sample: Landscape
Video Caption Data Sample: Sports
Video Caption Data Sample: Lifestyle