Text-to-Video (T2V) technology is a revolutionary innovation in the next generation of short video production. It not only provides unprecedented flexibility for the creation and expression of video content but also significantly simplifies the video production process. Text-to-Video technology is based on the latest developments in deep learning and natural language processing (NLP). This technology combines image and video generation models, such as transformer-based models, with the ability to understand text, enabling the transformation of text into video frames.
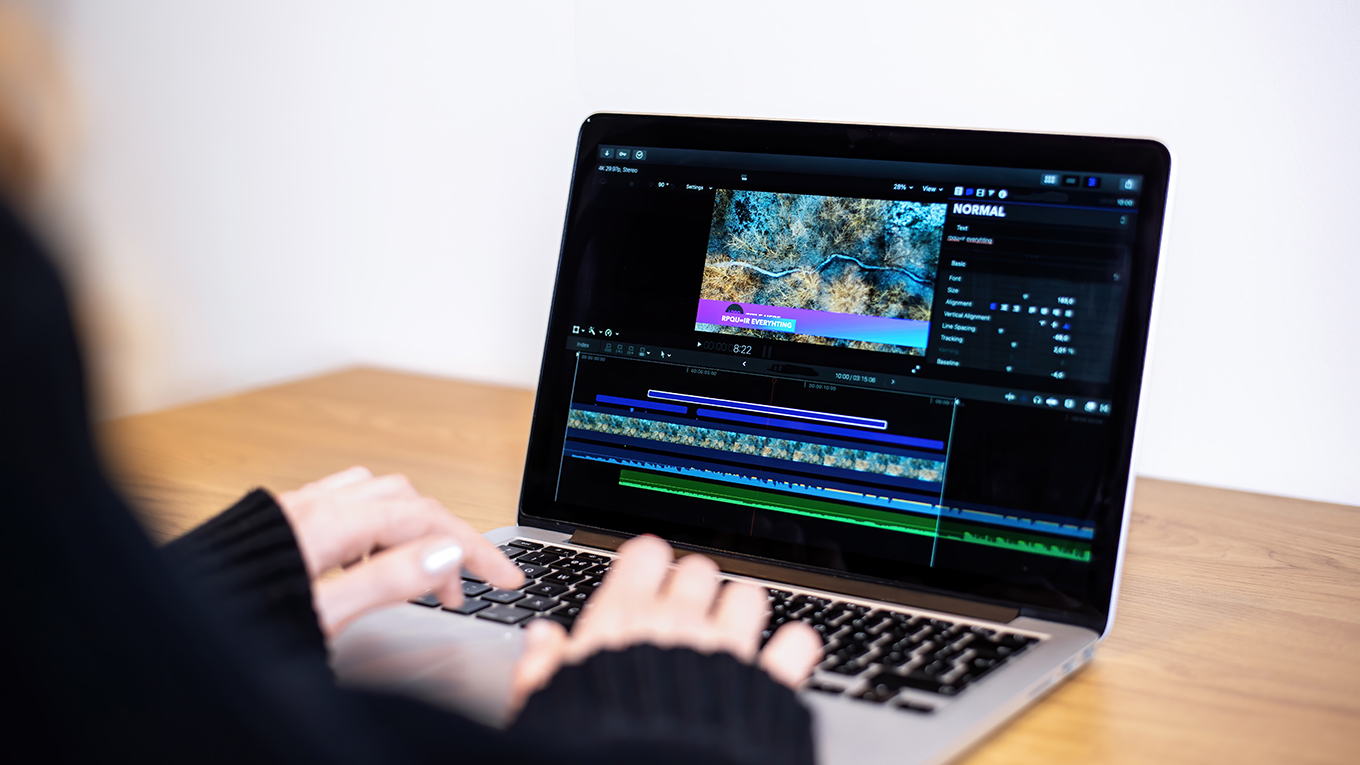
Text-to-Video systems can comprehend input text content and generate videos based on this information, including understanding elements like scenes, actions, emotions, etc. Users can customize various aspects of the video, such as style, color, sound effects, to fit different brand and storytelling requirements. Compared to traditional video production, Text-to-Video technology greatly improves production efficiency and lowers the technical barriers. Currently, tools like Shortmake AI, Pika Labs, RunwayML’s Gen2, and Li Feifei’s team’s latest achievement W.A.L.T are actively applying such technologies in the market.
Applications of Text-to-Video
– Shortmake AI
Shortmake is a tool that utilizes artificial intelligence technology to simplify the process of video creation and editing. It is designed as a revolutionary tool to assist users in easily creating engaging videos with viral potential. The key features of Shortmake include:
• AI-Driven Editing: Shortmake employs artificial intelligence technology to streamline the video creation and editing process.
• Rapid Production of Short Videos: The tool is designed to help users quickly produce attractive short video content.
• Unlimited Creativity: Users have the freedom to unleash their creativity, making it suitable for various types of video content.
The platform first generates a detailed scene description based on a short user input, and then synthesizes a short video based on this detailed scene description.
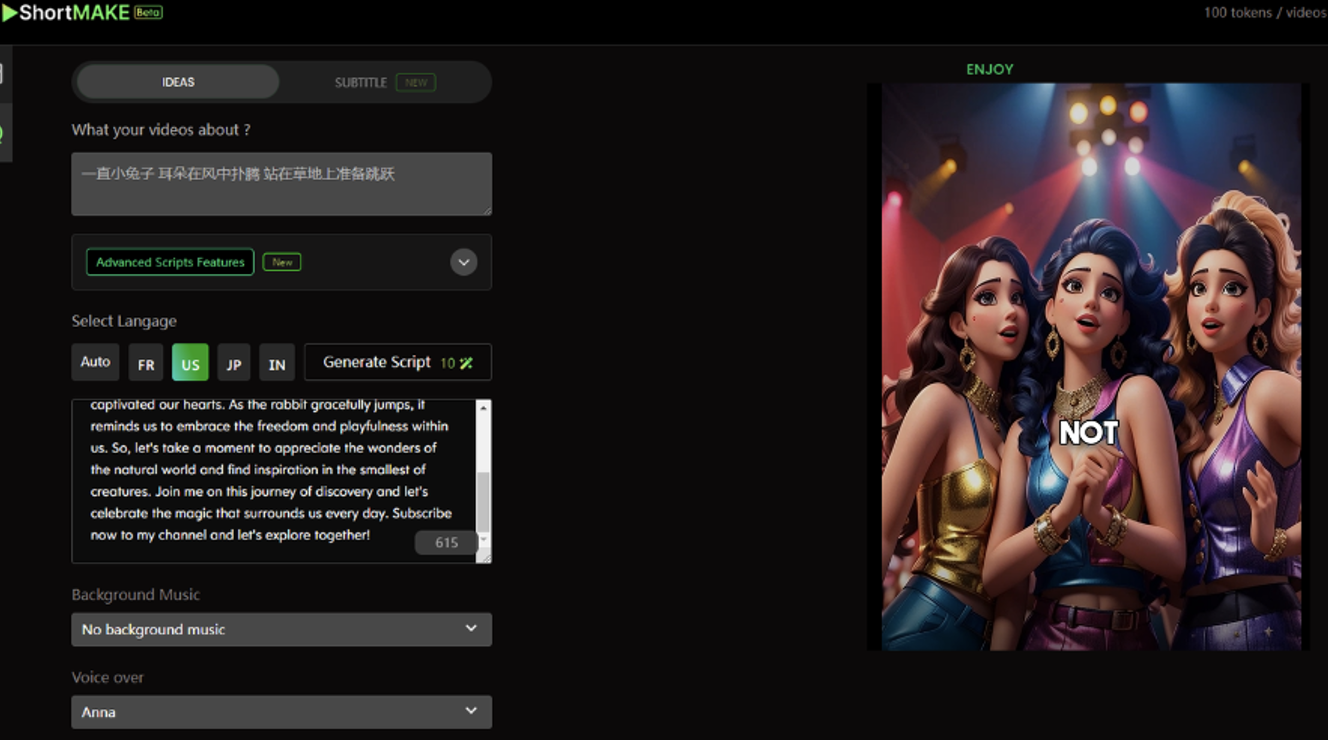
– Pika
Pika Labs is a company dedicated to developing an AI text-to-video platform. Users can input text or provide an image and have it animated to generate a video. Pika Labs can produce exceptionally smooth videos, suitable even for advertising and film production. The company’s AI excels in temporal consistency and transitions, resulting in very fluid video effects. Currently, the Pika Labs official website is in a waiting trial phase, but the effects of Pika can be experienced on the Discord social platform. Overall, the generated effects are quite satisfactory.
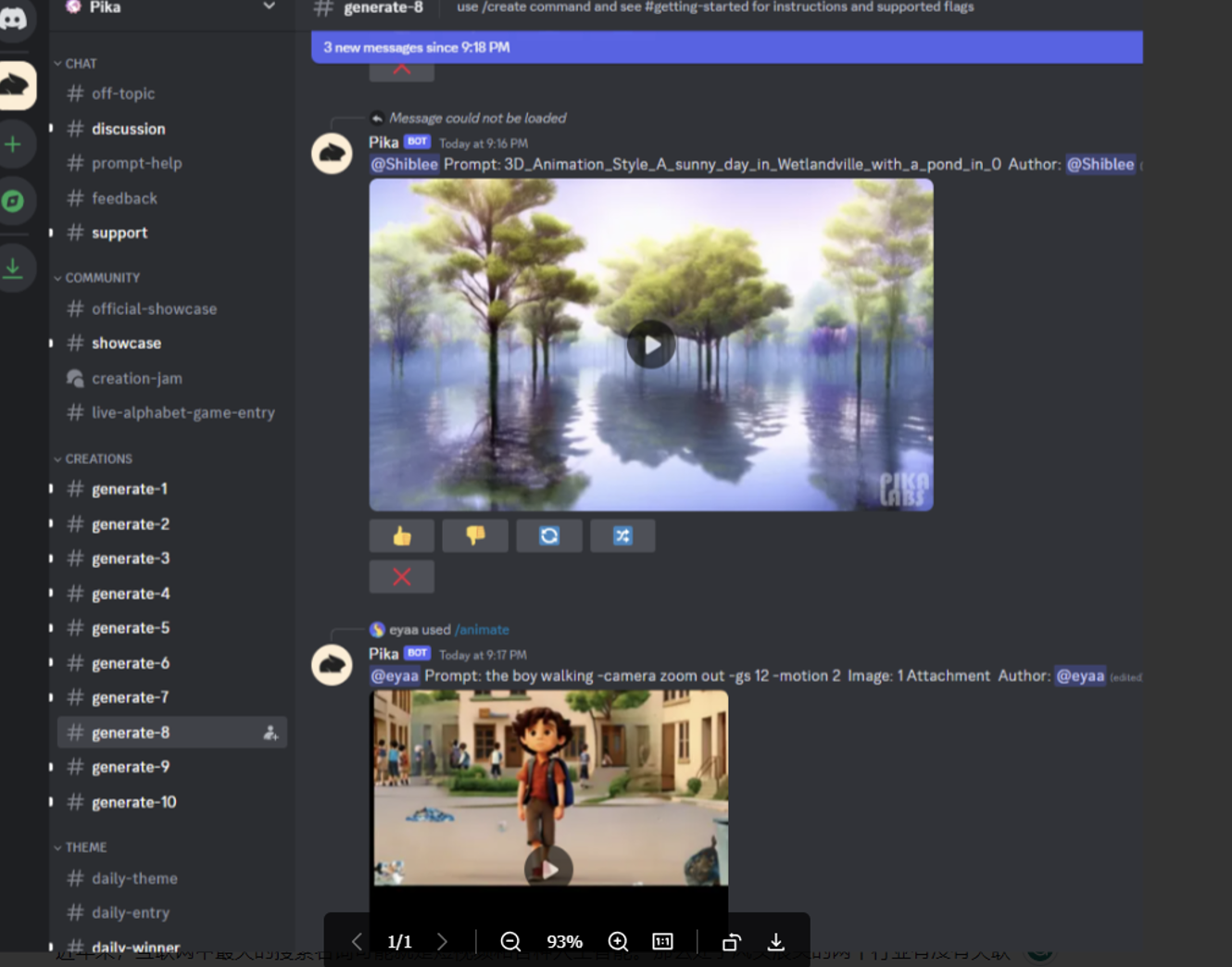
– RunwayML’s Gen2
AI startup Runway, supported by Google, has developed an AI image generator called Stable Diffusion. In early June, they released the Gen-2 model, which is capable of generating videos based on text s or existing images. Previously, Gen-2 was only available to a limited waiting list of users, but now it has been opened up for all users. Runway currently offers approximately 100 seconds of free video generation, with each video lasting about 4 seconds.
Similar to many generative AI models, characters in the videos created by Gen-2 may appear extremely unnatural and unrealistic. However, aside from the characters, videos featuring landscapes and objects are highly impressive. Below is a video of KTV singing that I generated using Runway, and the video scene is very clear and high-fidelity.
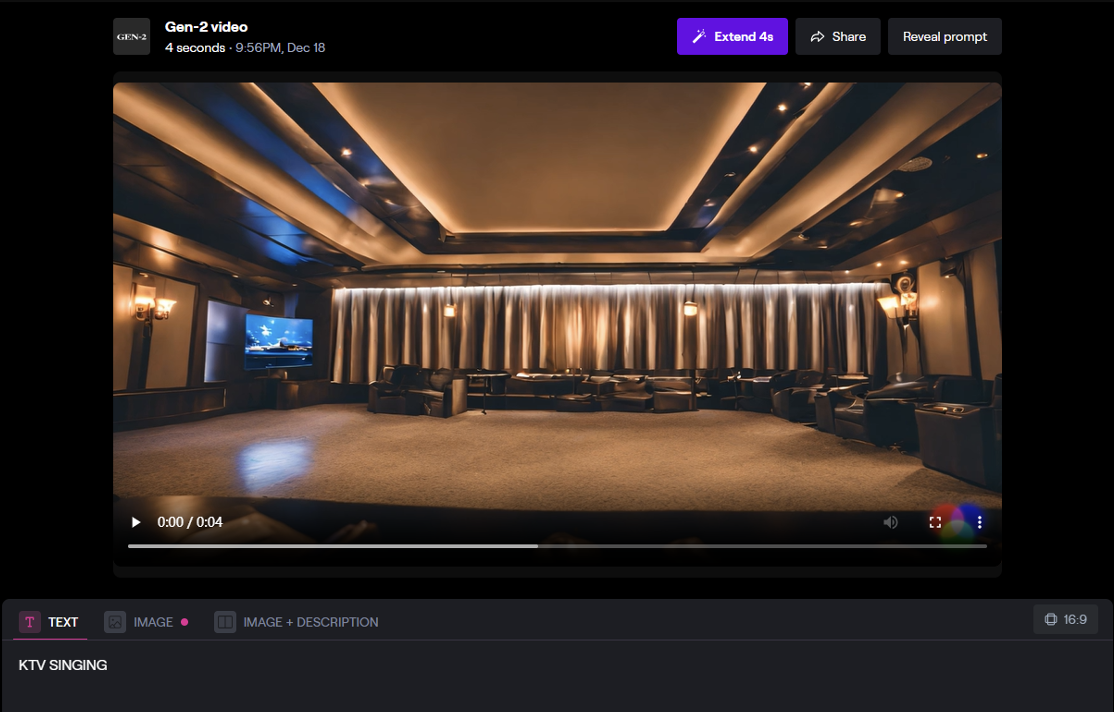
– W.A.L.T
Recently, Li Feifei and their team proposed a framework called W.A.L.T for text-to-video generation. This is a realistic video generation method based on Transformer. It primarily adopts two strategies: first, it uses a causal encoder to handle images and videos in a unified latent space, achieving cross-modal training and generation; second, it employs a customized attention mechanism for spatiotemporal modeling to enhance memory and training efficiency. In addition, they have developed three text-to-video generation models, including a basic video diffusion model and two super-resolution models capable of generating 512×896 resolution videos at 8 frames per second.
W.A.L.T. github: https://walt-video-diffusion.github.io/
W.A.L.T. paper: https://walt-video-diffusion.github.io/assets/W.A.L.T.pdf
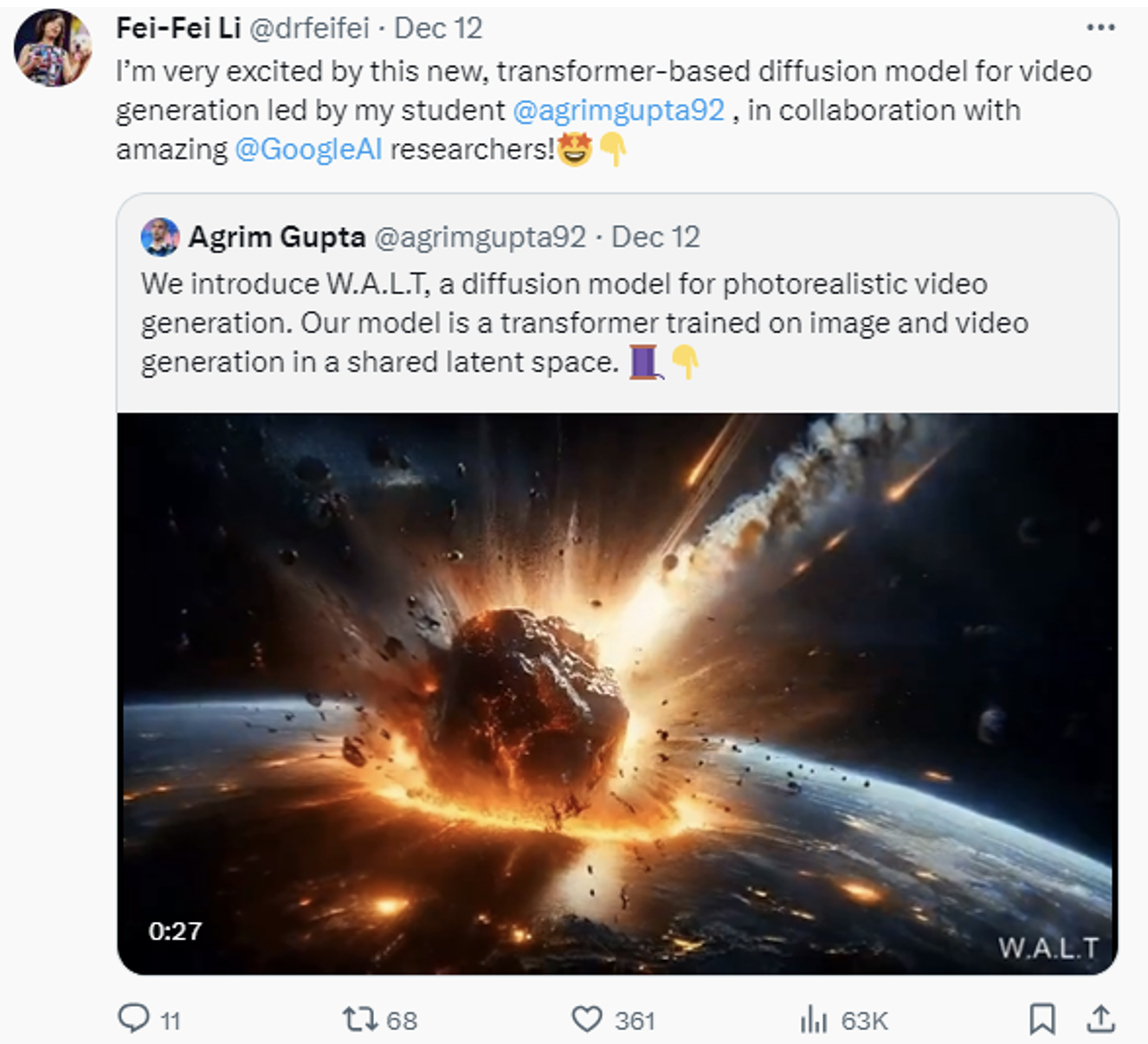
Core Technology of Text-to-Video Synthesis
Text-to-Video synthesis is an innovative AI technology that turns text descriptions into dynamic videos. Key components include Natural Language Processing (NLP) for text interpretation, deep learning and neural networks (e.g., GANs, VAEs) for realistic video generation, and temporal modeling (RNNs, LSTMs) for video coherence. Computer vision aids in understanding video content, incorporating 3D scene reconstruction, object detection, and motion analysis. This transformative technology finds applications in film, advertising, virtual reality, and education, offering creative possibilities for designers. Implementation requires ample training data and computational resources, with large annotated datasets and GPU acceleration essential for processing complex neural networks and producing high-quality video content.
Datasets for Text-to-Video
The implementation of Text-to-Video indeed demands a substantial amount of training data, along with appropriate data types and forms, to ensure the generation of high-quality video content. Here are the required forms of training data for Text-to-Video:
• Text Data: Firstly, you need a large-scale text dataset that encompasses various text content, such as descriptions, storylines, dialogues, etc. This text data should be diverse to ensure the model can handle video generation requests of different themes and styles.
• Video Data: In addition to text data, you also need video data associated with the text. This can include actual videos related to the text content and synthetic videos where the text’s content has been transformed into video frames. This video data should cover a variety of contexts, scenes, and themes to enable the model to learn different types of video generation.
• Multimodal Data: Text-to-Video is a multimodal task, involving the processing of both text and video data types. There needs to be relative alignment between text and video labels in the dataset, allowing the model to learn how to transform text into corresponding video content.
In summary, the training data for Text-to-Video needs to consider the multimodality of both text and video, ensuring diversity and coverage in the data, as well as detailed annotation to aid the model in learning the mapping relationship from text to video. Additionally, since models typically require extensive parameters and computational resources, a sufficiently large dataset is necessary to train these complex neural networks.
Short video creators use innovative expressions and creative content to engage audiences, including the use of various filters, effects, and music. This fosters diversity and innovation in video content. Text-to-Video can better assist creators in organizing creative ideas intelligently and synthesizing videos in a more automated manner.