At this year’s Paris Olympics, approximately 10,000 athletes from over 200 countries and regions will compete with determination for the Olympic spirit and their dreams. “AthleteGPT,” a powerful intelligent voice assistant, will assist athletes and staff from different countries and regions, enabling smoother communication and interaction throughout this summer event.
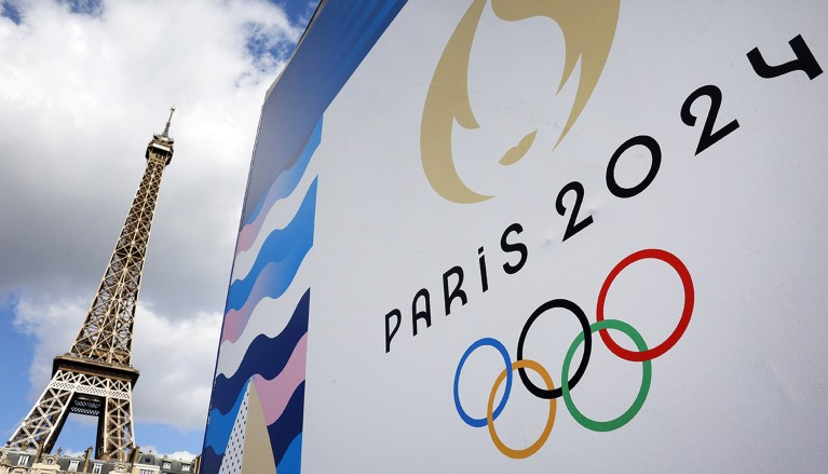
How do I get to the sports venue? Can I watch the opening ceremony on live? Where can I collect gifts provided by sponsors? These are the kinds of questions that athletes may have, and they will be answered in detail by the AI assistant AthleteGPT, which helps approximately 11,000 athletes of different languages and cultures navigate the venues and follow the rules and guidelines. AthleteGPT is developed by Mistral AI and uses Intel® Gaudi® AI deep learning processor. It is capable of “quickly browsing through millions of pages of information and answering questions around the clock.”
AthleteGPT Core Function- Automatic Speech Recognition
More Natural and Convenient Interaction: Athletes do not need to manually enter questions or commands; they can easily obtain the required information just by speaking. This is especially important for athletes who are unable to operate devices such as mobile phones during training or competition breaks.
Overcoming Language and Cultural Barriers: By supporting multiple languages and accents, AthleteGPT enables athletes from around the world to use it without barriers, achieving seamless cross-language communication.
Enhancing Interaction Efficiency and User Experience: With fast and accurate speech recognition, AthleteGPT can instantly respond to athletes’ inquiries, reducing waiting time. Real-time voice responses make the user experience smoother and more natural.
Multilingual speech recognition dataset plays an essential role
AthleteGPT is capable of understanding and processing voice inputs from athletes around the world who speak different languages, including various dialects and accents. It provides information in multiple languages quickly and accurately, thanks to the training data from multilingual speech recognition datasets.
First, collect speech data from various countries and regions, then apply noise reduction to enhance audio quality, and finally segment and label the data for model training.
These datasets need to encompass not only the standard pronunciations of major international languages but also samples of different dialects and accents to improve the accuracy and robustness of the speech recognition model.
Dataocean AI‘s Multilingual Speech Corpus for Speech Foundation Models
As companies expand their global operations, the demand for and performance expectations of multilingual and multi-speaker speech recognition models are increasing. The performance of these models is constrained by factors such as the diversity and scale of the speech data, the inclusion of a sufficient number of languages and speakers, and the accuracy of the labeling.
Dataocean AI has launched an intelligent speech dataset of over 100 languages, covering a total of 103 languages, including 259,672 hours of voice data and voice samples from 215,891 speakers, meeting the diverse needs of clients for various languages.
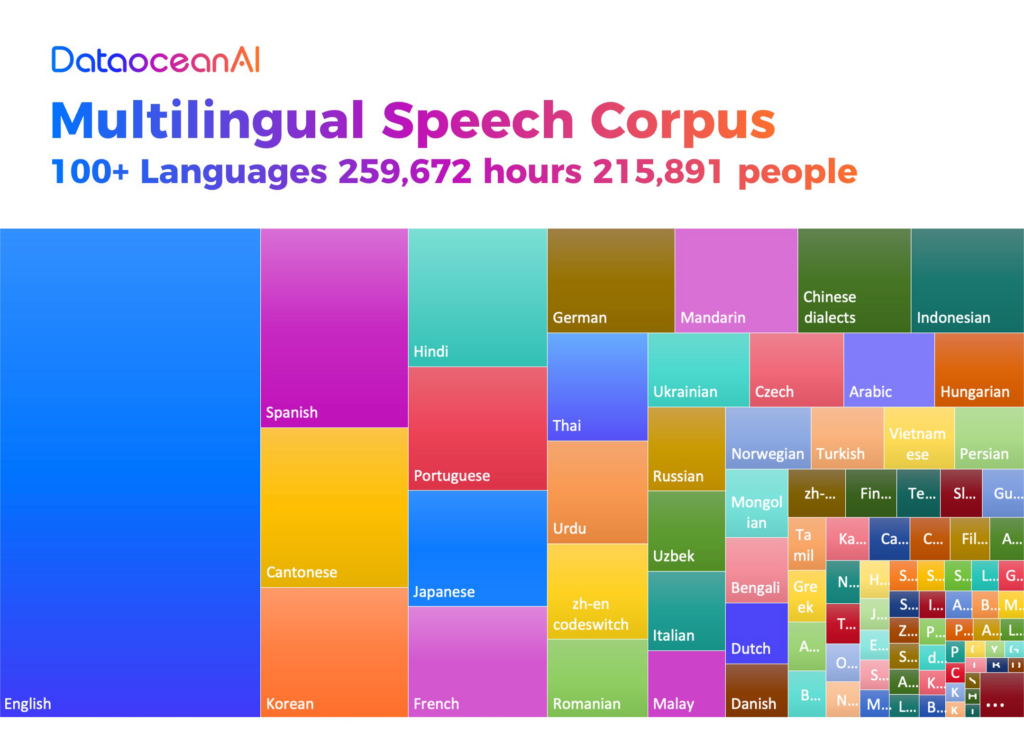