Audio-visual speech recognition has received a lot of attention due to its robustness against acoustic noise. Recently, the performance of automatic, visual, and audio-visual speech recognition (ASR, VSR, and AV-ASR, respectively) has been substantially improved, mainly due to the use of larger models and training sets. The authors in this paper proposed to use publicly available pre-trained automatic speech recognition (ASR) models to automatically transcribe unlabelled datasets, which are subsequently employed to augment the training data of audio, visual, and audio-visual (ASR, VSR, AVSR) models.
Methodology
The proposed Auto-AVSR comprises two steps as shown in Fig. 1. The first is a label-generation pipeline, where the audio stream from the unlabeled audio-visual data is fed into a pre-trained ASR model to produce transcriptions. The second is an AVSR model comprising a visual encoder for VSR, an audio encoder for ASR, and shared decoder and CTC modules. Here, the VSR front-end is based on a modified ResNet-18, where the first layer is a spatiotemporal convolutional layer with a kernel size of 5×7×7 and a stride of 1×2×2. The temporal back end, which follows the front end, is a Conformer encoder module. Similarly, the ASR encoder consists of a 1D ResNet-18 followed by Conformer layers. The ASR and VSR encoder outputs are fused via a multi-layer perceptron (MLP) before being fed into the shared CTC and Transformer decoder modules.
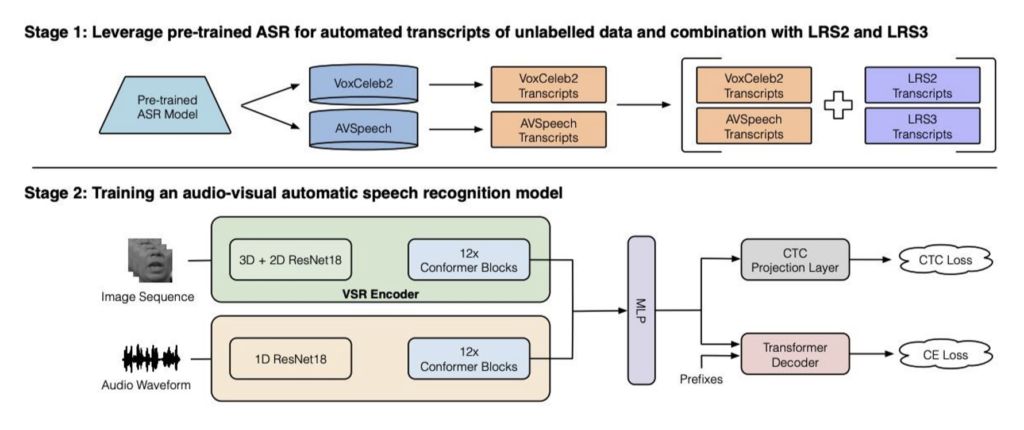
Figure 1. AV-ASR architecture overview. In the first stage, a pre-trained ASR model is leveraged to produce automatically generated transcriptions for unlabelled audio-visual datasets. Then, these unlabelled datasets are combined with the labeled training sets, including LRS2 and LRS3, for training. The frame rate of audio and visual features from the ASR and VSR encoders is 25 frames per second (fps).
Do better Librispeech ASR models provide better transcriptions for VSR?
The authors investigate the performance of several pre-trained ASR models including a state-of-the-art Conformer-Transducer model pre-trained on LibriSpeech, HuBERT, wav2vec 2.0, and Whisper. The results shown in Table 1 indicate that pre-training the ASR model on larger data does not always lead to higher VSR and ASR performance.
Impact of the number of hours of unlabelled data used
Table 2 shows the impact of varying the number of hours of unlabelled data on the performance of ASR and VSR models on LRS3. The results imply that augmenting the training data with more data which are automatically transcribed from unlabeled data results in higher performance on both LRS2 and LRS3 datasets. In addition, the authors suggest that the improvement for the ASR model is marginal when using more than 1 578 hours of unlabelled training data, indicating that the ASR performance may have saturated.
Comparison with the SOTA
The authors compared the proposed method with state-of-the-art performance with respect to WER. For LRS2, it is clear that the proposed visual-only, audio-only, and audio-visual models further push the state-of-the-art performance to a WER of 14.6 %, 1.5 %, and 1.5 %, respectively. For LRS3, the visual-only model trained via the proposed approach has a WER of 19.1 %, which is outperformed only by the ViT3D-CM system (17.0 % WER) which uses 26 times more training data.
Robustness to noise
The robustness of the proposed method to noise is discussed in this section. The results show that the performance of audio-only models is close to the audio-visual counterpart in the presence of low levels of noise, whereas the performance gap becomes larger as the noise levels increase.
Conclusion
The paper proposed a simple and efficient method that can scale up audio-visual data for speech recognition by automatically transcribing unlabeled video data via a pre-trained ASR model. The authors suggest that including more unlabeled data through the use of the proposed method leads to higher performance for ASR, VSR, and AVSR. In addition, the proposed audio-visual model is more robust against noise than its audio-only counterpart.
The CNVSRC 2023 Challenge
To promote the development of AVSR techniques and contribute to the AVSR community, we co-organized the Chinese Continuous Visual Speech Recognition Challenge 2023 (CNVSRC) with Tsinghua University, Beijing University of Posts and Telecommunication, and Speech Home in NCMMSC 2023.
We provide three datasets for participants to train, validate, and evaluate their systems. CN-CVS is the largest open-source Mandarin audio-visual and is used as the training data for the close track. CNCSRC-Single and CNVSRC-Multi are employed as the development and test sets in single-speaker and multi-speaker ASRV tasks, respectively. Here, CNVSRC-Single comprises more than 100 hours of single-speaker video data collected from the internet, while CNVSRC-Multi contains reading speech and public speech.
The reading data in CNVSRC-Multi comes from the dataset. This dataset was donated to CSLT@Tsinghua University by Beijing Haitian Ruisheng Science Technology Ltd.4 to promote scientific development.
CNVSRC 2023 Register:http://cnceleb.org/competition
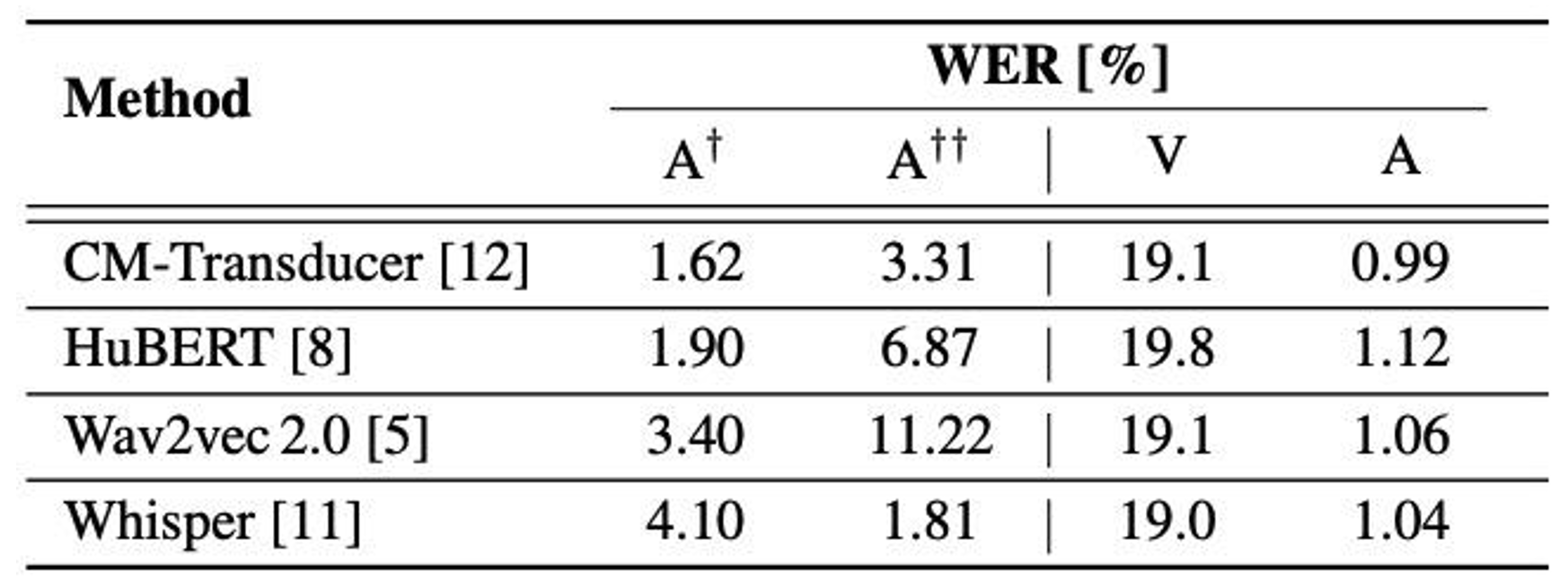
Table 1. Impact of the pre-trained ASR models used to generate automatic transcriptions from unlabelled data on the performance of VSR/ASR models on the LRS3 dataset. † and †† denote the word error rate (WER) reported on the LibriSpeech test-clean set and LRS3 test set, respectively. “CM” denotes Conformer. “V” and “A” denote the visual-only and audio-only models trained on LRW, LRS2, LRS3, VoxCeleb2, and AVSpeech (using the automatically-generated transcriptions from the corresponding pre-trained ASR model), with a total of 3 448 hours.
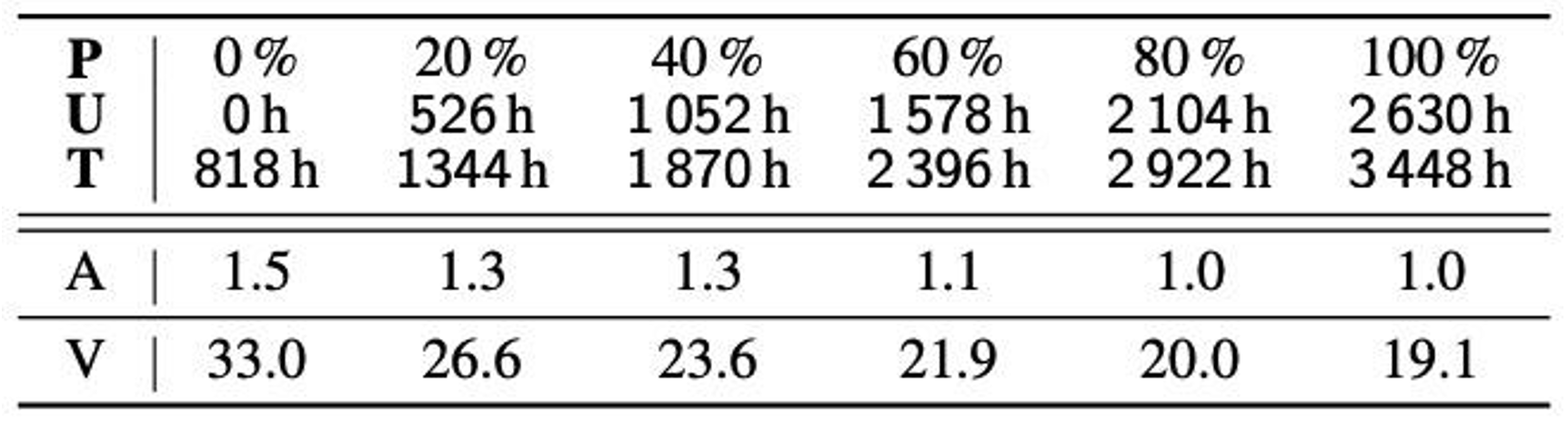
Table 2. Impact of the size of additional training data (from AVSpeech and VoxCeleb2) on the WER (%) of audio-only and visual-only models evaluated on LRS3. All models are initialized from a model pre-trained on LRW and trained on LRS2, and LRS3 plus X % hours of VoxCeleb2 and AVSpeech. “P” and “U” denote the amount of additional data in percentages and hours, respectively. “T” denotes the total amount of training data (hours).
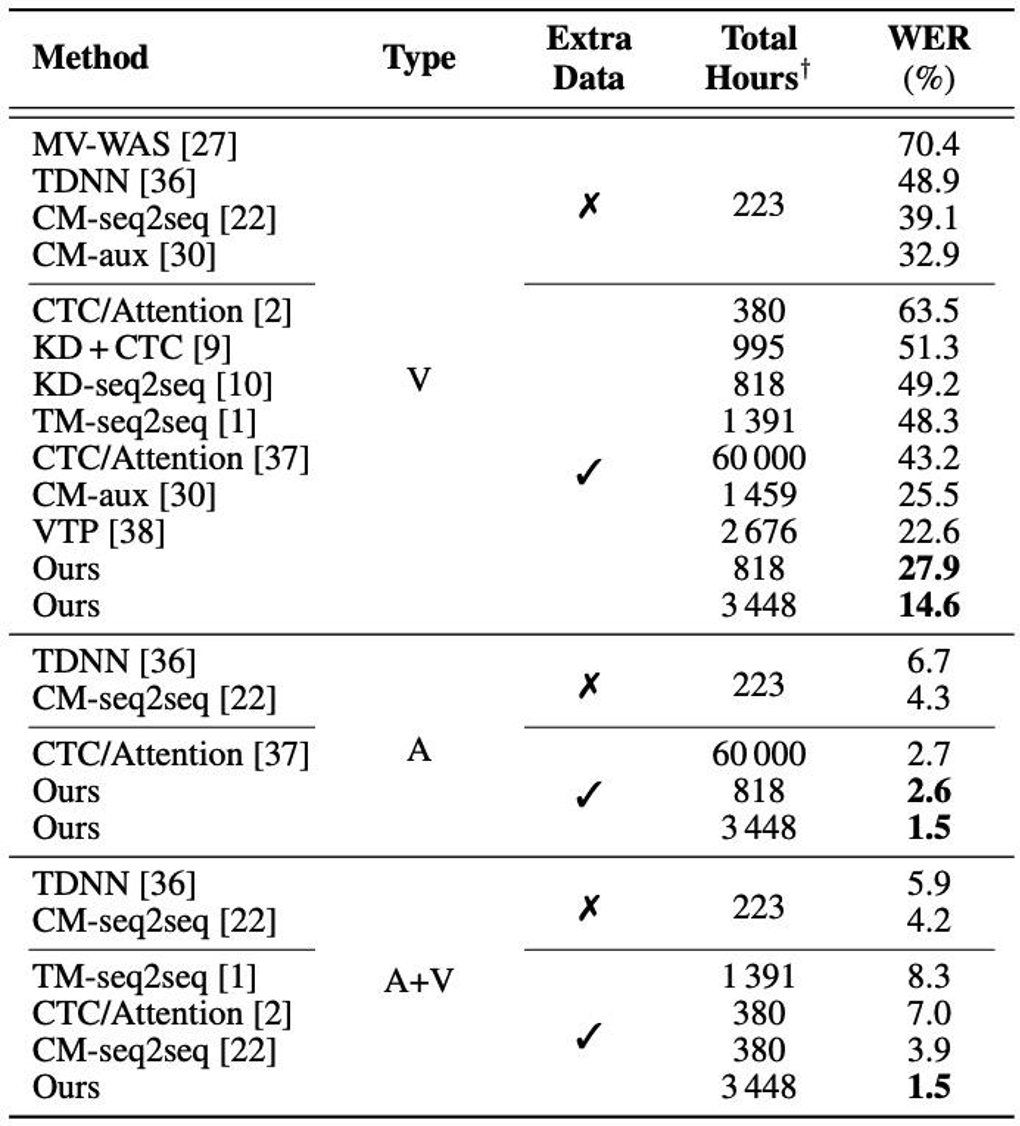
Table 3. WER (%) of the proposed audio-only, visual-only, and audio-visual models on the LRS2 dataset. † The total hours are counted by including the datasets used for both pre-training and training. The proposed model trained on 818 hours uses LRW, LRS2, and LRS3. Our model trained on 3 448 hours uses LRW, LRS2, LRS3, VoxCeleb2, and AVSpeech.
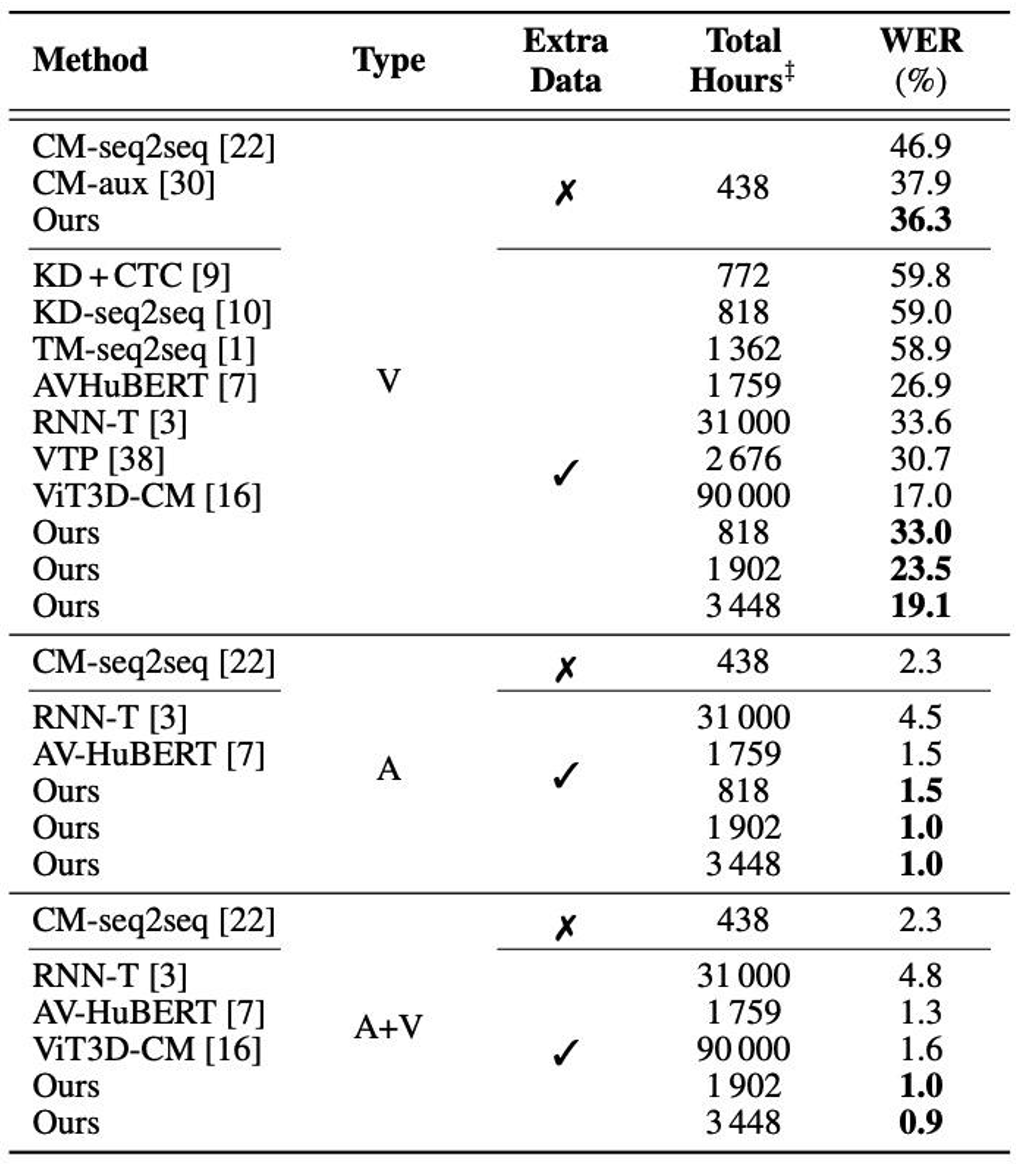
Table 4. WER (%) of the proposed audio-only, visual-only, and audio-visual models on the LRS3 dataset. ‡ The total hours are counted by including the datasets used for both pre-training and training. Our model trained on 818 hours uses LRW, LRS2, and LRS3. Our model trained on 1 902 hours uses LRW, LRS3, and VoxCeleb2. Our model trained on 3 448 hours uses LRW, LRS2, LRS3, VoxCeleb2, and AVSpeech.
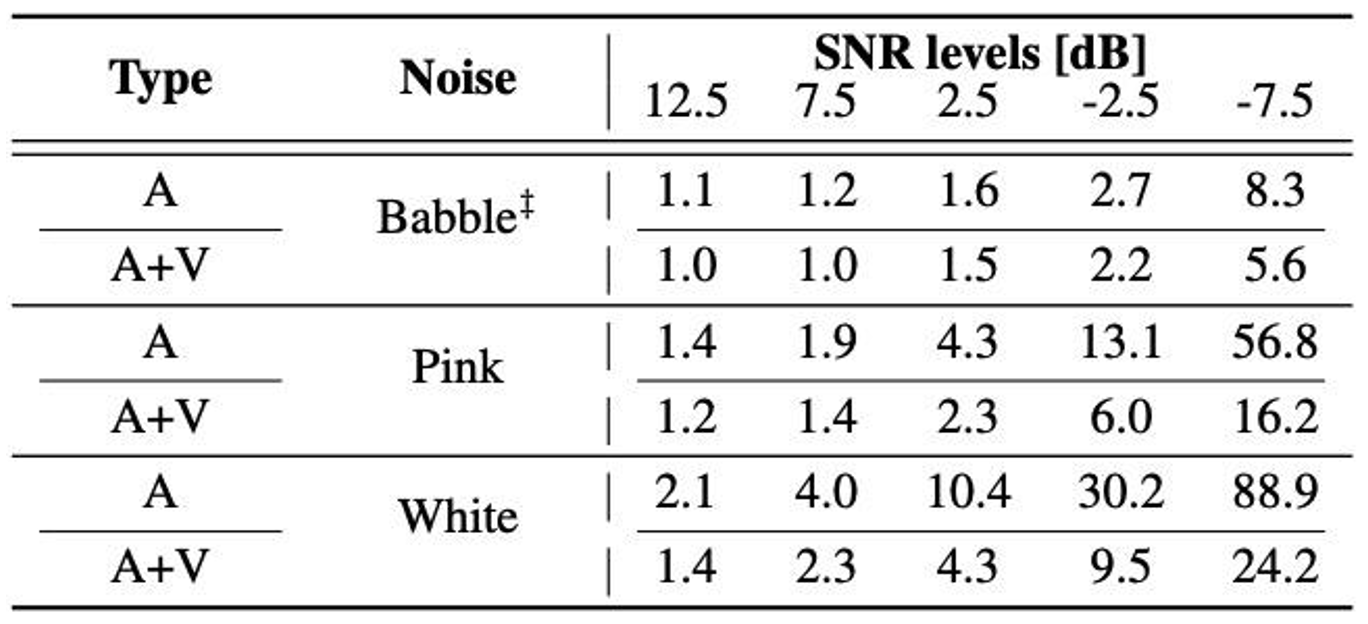
Table 5. WER (%) of the proposed audio-only and audio-visual models as a function of the noise levels on the LRS3 dataset. The babble noise from the NOISEX dataset is used for training while one of the SNR levels from [-5 dB, 0 dB, 5 dB, 10 dB, 15 dB, 20 dB, ∞ dB] is selected with a uniform distribution. For testing, the pink and white noise from the Speech Commands dataset is added to the raw audio waveforms with a specific SNR level. ‡ denotes the noise type used in both training and test sets.
*Paper: Auto-AVSR: Audio-Visual Speech Recognition with Automatic Labels
https://ieeexplore.ieee.org /10096889