Large language models have gradually become a part of our work, study and entertainment. In addition to the familiar ChatGPT, there are multi-modal large models such as LLaVa and ImageBind-LLM, which respond to our needs 24/7 to answer and answer questions. Most of the answers fed back by these large models are accurate and useful, but when you use them, you will inevitably encounter their ‘serious nonsense’.
For example, when I asked ChatGPT below to give me five references for modeling figure skating equations with polynomials, four of the five references given by ChatGPT were fabricated and did not exist.
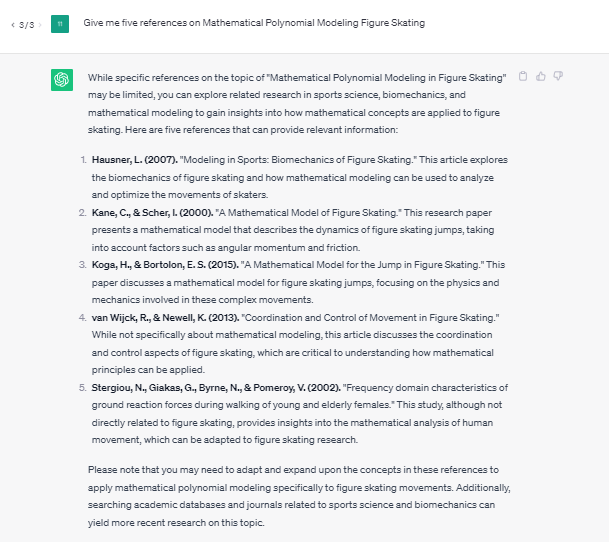
What’s up with the nonsense of models like ChatGPT? What is this phenomenon?
——This is AI Hallucination.
AI Hallucination refers to the completely fabricated information generated by the AI model, information that is not real, and inaccurate information.
The term ‘hallucination’ is used because of its similarity to the human psychological phenomenon in which a person perceives something that does not exist in reality. Just like when we look at this picture, it seems to be moving, but it is not actually moving.
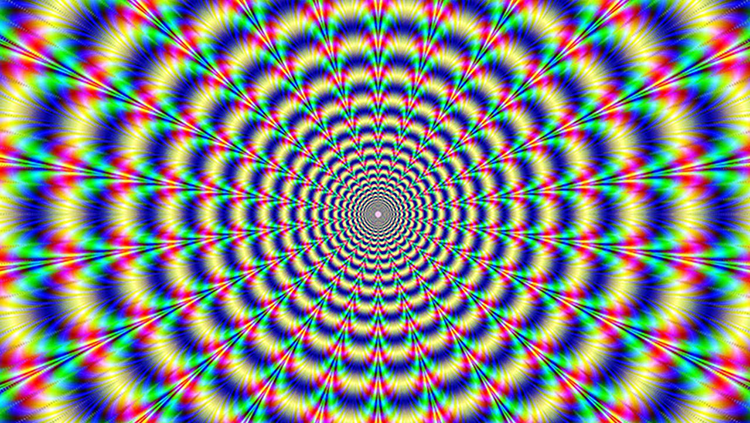
Why AI Hallucinates?
‘Even state-of-the-art models are prone to falsehoods, which exhibit a tendency to fabricate facts in moments of uncertainty,’ the OpenAI researchers said in the report. These hallucinates are especially problematic in domains that require multi-step reasoning, because a logical A mistake is enough to destroy a larger solution. So, what are the causes of AI Hallucinations?
- Dirty Training Data
Outdated or low-quality training data misleads AI models during training, leading to inaccuracies during inference. If the data used to train the AI is not current or of poor quality, the AI may make hallucinatory decisions based on inaccurate information.
In addition, incorrect classification or labeling of data is another reason. AI can misinterpret information if data is not properly classified or labeled, leading to hallucinations.
If there are errors, inconsistencies, or biases in the training data, the AI can be affected by these issues and produce spurious results.
- Model Flaws
If overfitting occurs during training, AI Hallucinates will also appear for the user’s new input reasoning. When an AI model is overfitting the training data, it can start generating output that is too specific to the training data and doesn’t adapt well to new data. This can cause the model to generate spurious or irrelevant output.
At the same time, the model may not fully understand the context. AI models that lack an adequate understanding of context may generate out-of-context or irrelevant output. This can also cause the model to generate spurious or nonsensical results.
- Spoofing
Unlike traditional ‘offensive and defensive’ contests, AI models are also vulnerable to adversarial attacks. When a malicious attacker intentionally tampers with a model’s inputs, it can cause it to generate incorrect or malicious outputs. At present, there are many spoof and antispoof schemes competing with each other in academia.
Solutions to the AI Hallucinates
‘Detecting and mitigating logical errors or hallucinations in a model is a critical step in building consistent general artificial intelligence (AI),’ Karl Cobbe, a researcher at OpenAI’s Mathematical Thesis Generator (Mathgen), said in an interview. AI Hallucinates, we can adopt the following measures.
- AI Data Control
Data is the cornerstone of AI models. It is crucial to ensure the high quality of training data, which includes taking multiple measures to maintain and improve the quality level of the data to ensure that the AI system can produce accurate and reliable results.
First, it is crucial that data is updated in a timely manner to reflect the latest information. Because data is constantly updated, using outdated data can lead to misleading results for AI systems. Therefore, regular updates to the data to reflect the latest information and changes are critical to maintaining model accuracy.
Second, careful classification and labeling of data helps reduce the probability of misclassification or labeling. This requires focusing on the details of the data and applying strict standards to ensure that the data is correctly classified and labeled.
The meticulous classification and labeling process helps provide high-quality training data and reduces the risk of hallucinations in the AI system. You can use high-quality and accurate data annotated by data companies to fine-tune the model and guide the value orientation of the large model trained with big data.
- Algorithm ImprovementsI
Use Reinforcement Learning from Human Feedback (RLHF) methods, as OpenAI does. RLHF involves developing a reward model based on human preferences and feedback that will guide language models to provide more consistent outputs that are useful, honest, and harmless outputs.
An automatic error correction mechanism is introduced to reduce over-reliance on the quality of training data. These mechanisms are able to detect and repair errors or inconsistencies in the data, thereby improving the performance of algorithms in the face of irregular data.
Further, it integrates context understanding and context awareness functions to endow the algorithm with higher intelligence. This means that algorithms will better understand the background information and relevant context of the data, so that they can infer and process information more accurately.
Such integration makes the algorithm more suitable for dealing with complex problems and ambiguous data.
- Antispoofing
It is critical to develop detection and defense mechanisms against adversarial attacks that aim to reduce the impact of malicious attacks on AI models.
At the same time, the use of adversarial training methods can greatly improve the robustness of the model to resist potential attacks better.
First, adversarial attack detection and defense mechanisms are developed to protect AI systems from bad actors. These mechanisms are designed to identify and block malicious attacks, such as potentially deceptive information in input data or other forms of attack.
By implementing these mechanisms, AI systems are better able to discern and block malicious input, ensuring that the results they generate are accurate and trustworthy.
Second, using an adversarial training method is a strategy to enhance the robustness of the model. This approach forces the model to learn how to respond to potential attacks by intentionally introducing adversarial examples during training.
This training can help the model better understand and respond to bad inputs, reducing the risk of adversarial attacks. Through repeated exposure to adversarial situations, the model gradually becomes more robust and better able to deal with various potential attack vectors.
For the above solutions, the quality of training data is the top priority. DataOcean AI is committed to providing high-quality, large-scale, and precisely annotated structured data for large AI models.
In July, our Chinese 10-million-rounds Conversation Corpus DOTS-NLP-216 was released. The natural conversations are in line with Chinese natural expression habits collected under real scenes.
Fields covered multiple scenes like work, life, campus and multiple fields like financial, education, film and television, sports, automobiles, technology and so on. Helping enterprises to build high-quality generative AI applications.
Learn More About DOTS-NLP-216: Chinese Multi-Turn Dialogue Corpus – DataoceanAI