Recently, OpenAI released a multimodal voice and image upgrade of ChatGPT 4 called GPT-4V(ision). OpenAI unveiled a 19-page GPT-4V(ision) report titled “ChatGPT Can Now See, Hear, and Speak,” detailing information about the model.
This achievement means that ChatGPT can not only parse user input text but also has the capability to recognize and understand voice and images. Its voice recognition feature equips ChatGPT with skills similar to Siri. Additionally, ChatGPT offers different voice options for users to choose from and can convert voice audio into text or translate podcast content into other languages.
The development of GPT-4V(ision) was completed in 2022 and began offering early experiences in March 2023. The training strategy for GPT-4V is consistent with GPT-4, first using a large amount of text and image data for preliminary training, followed by fine-tuning through human feedback and reinforcement learning. By the end of September, OpenAI officially announced the multimodal upgrade of ChatGPT.
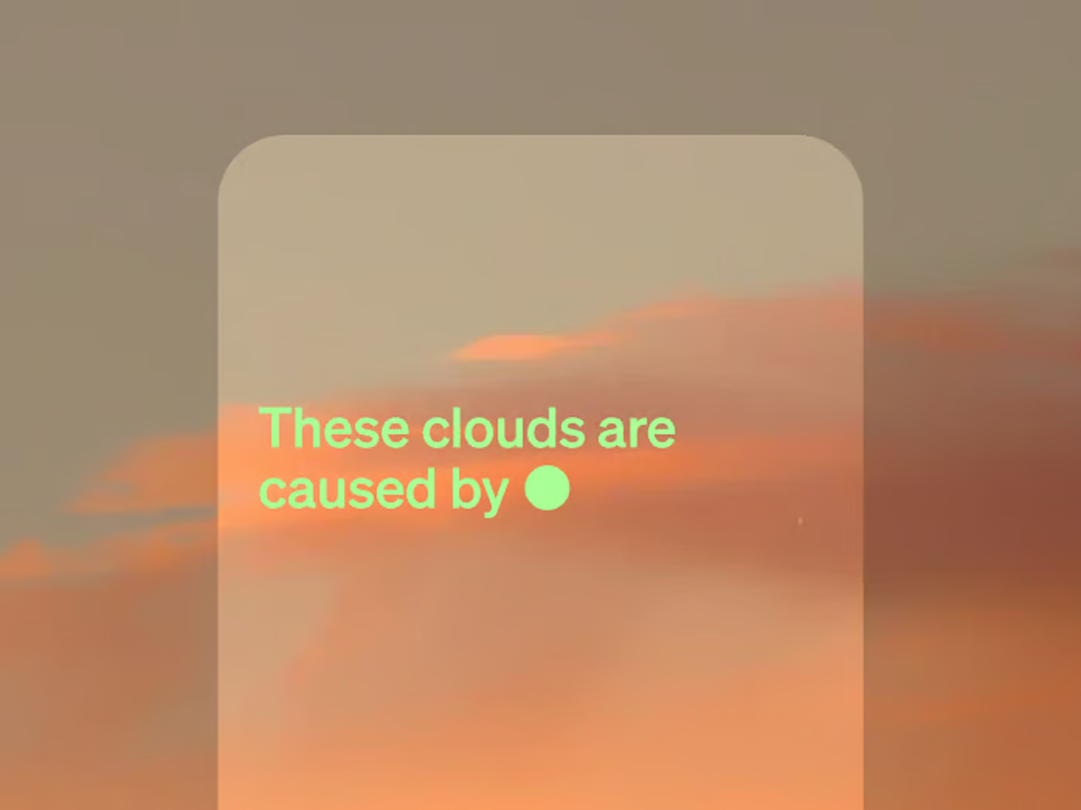
The main updates of the multimodal ChatGPT include:
• Image Analysis: The upgraded multimodal GPT3.5 and GPT4 can interpret the content in pictures and respond based on image information.
• Voice Processing: Voice recognition uses OpenAI’s Whisper technology. For speech synthesis, OpenAI adopts a novel “text-to-speech model”. Upon the release of this feature, users will have the option to activate it by configuring their app settings for voice interactions. They can choose from a selection of five distinct artificial voices, each endowed with unique names such as “Juniper”, “Sky”. OpenAI emphasizes that these voices have been meticulously curated in collaboration with seasoned voice actors.
Trying Out the Multimodal ChatGPT
Below is a case where ChatGPT describes an image:
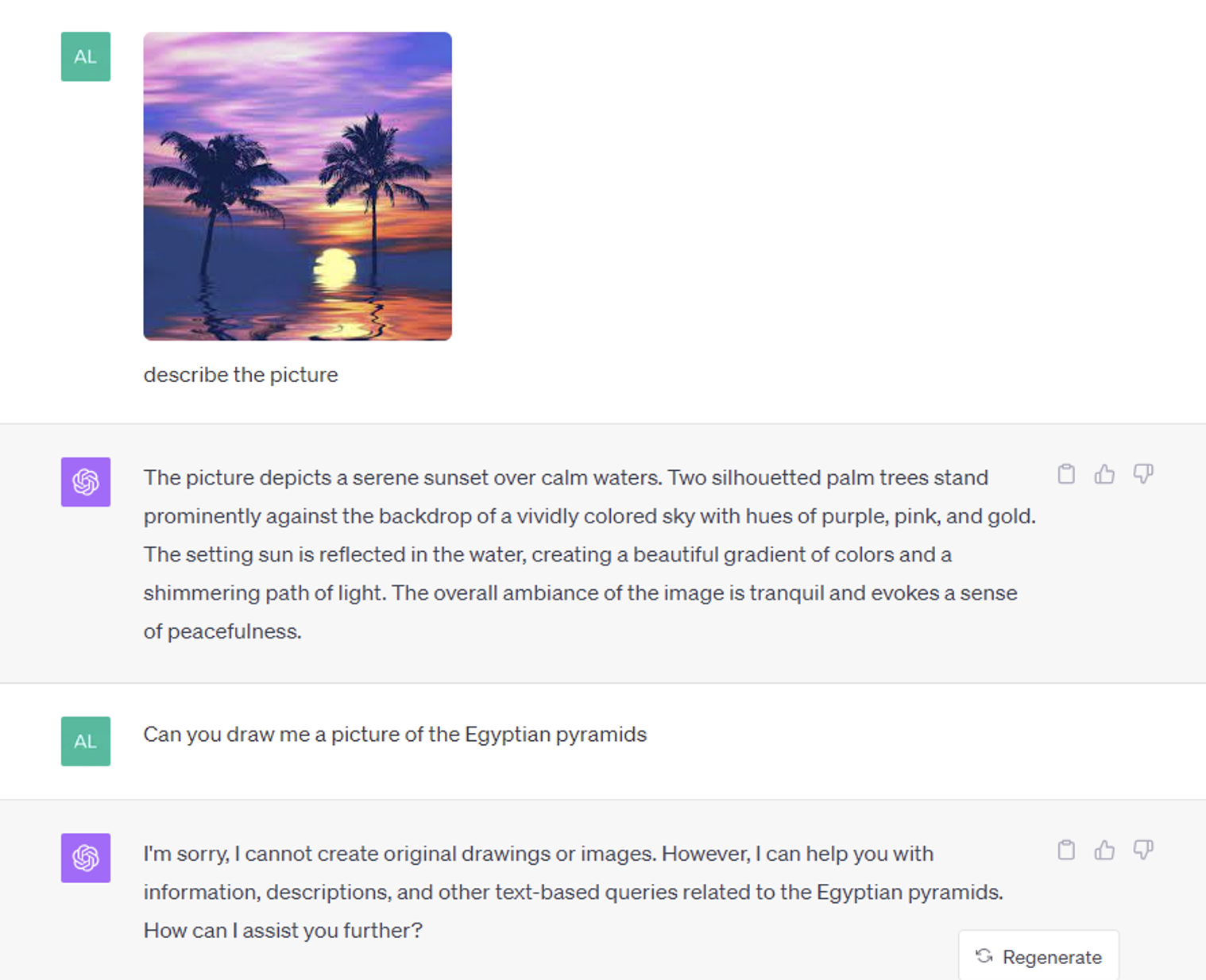
Converse with ChatGPT on the app, explaining the recent hot topic of superconductivity:
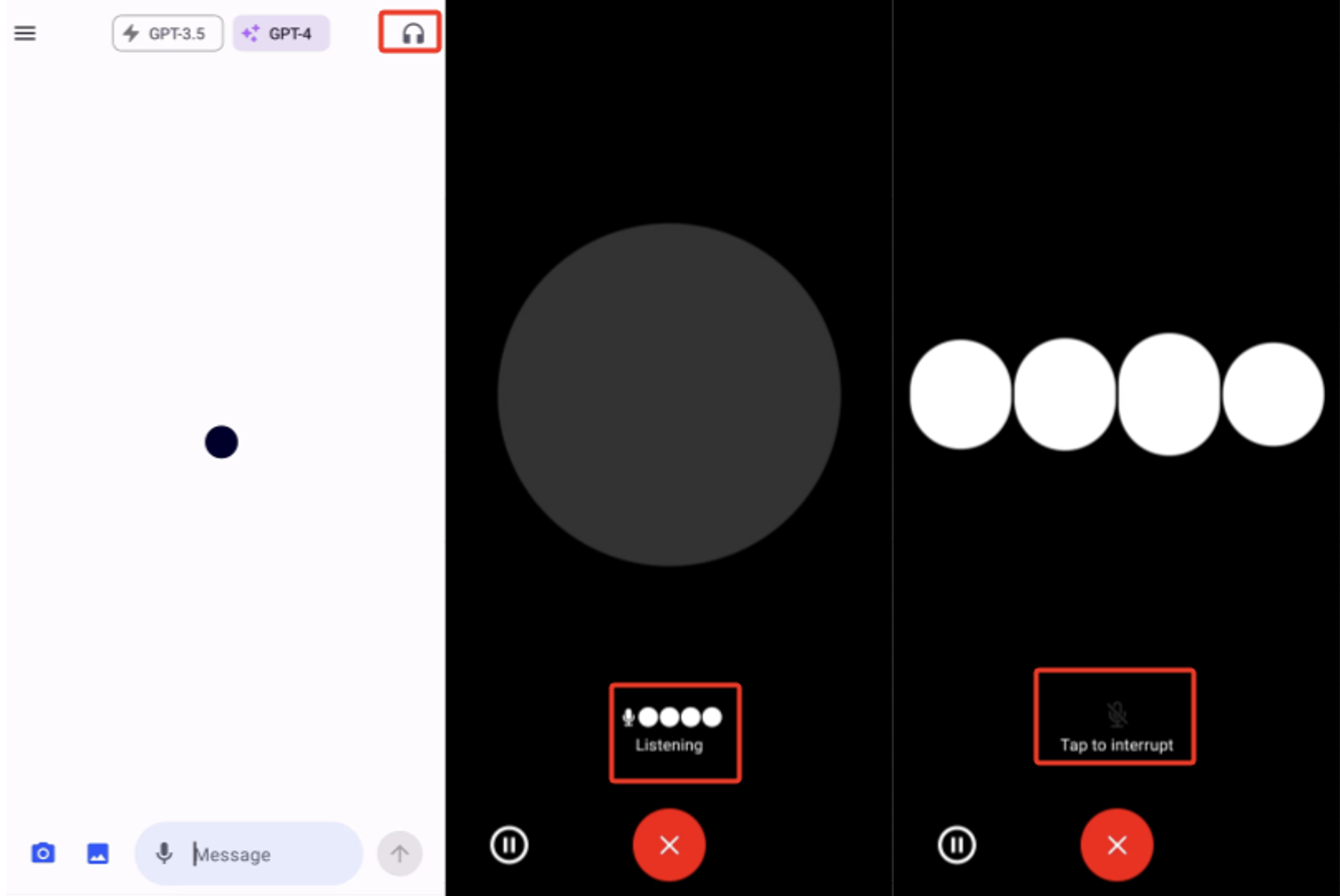
Below are the related real-time transcription subtitles:
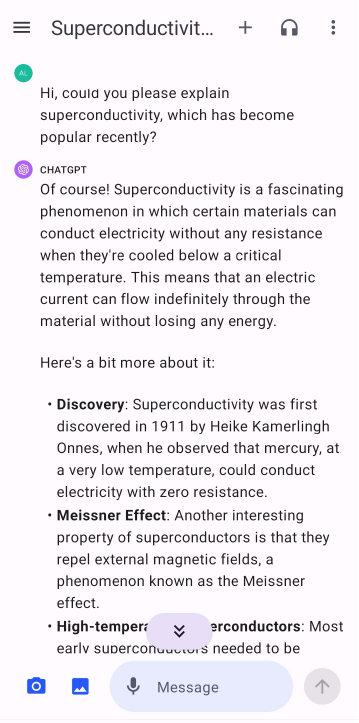
Its ability to understand and synthesize English voice is very smooth and accurate. Moreover, I also tried to communicate with it in Chinese, Japanese, and Korean, and found that it can understand and synthesize most languages. ChatGPT’s multilingual abilities are truly unrivaled by humans. I can only let it introduce the languages it knows:
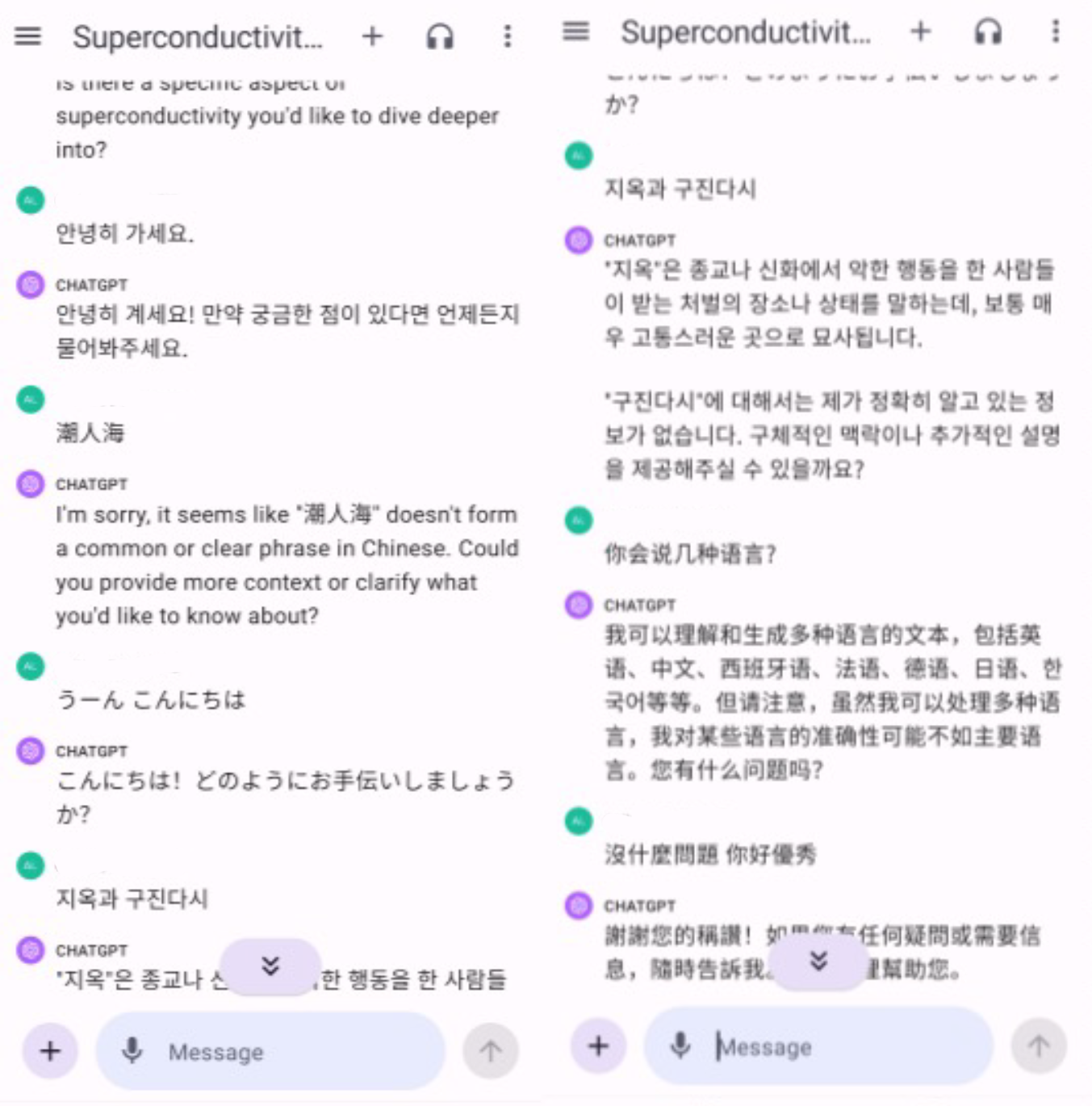
From his response, it’s evident that he knows many languages. However, compared to commonly seen languages with large data volumes like English and Chinese, there’s a gap in the recognition and synthesis accuracy for low-resource languages. This reflects a fact: the scale and quality of data determine the performance of an AI model. Due to the scarcity of data for low-resource languages, the uniqueness of pronunciation, and the difficulty of language annotation, ChatGPT’s voice recognition and synthesis for these low-resource languages are not very accurate.
How to Improve Further
ChatGPT is already very impressive, but there is room for optimization when it comes to low-resource languages, as mentioned above. OpenAI officially announced that their ASR model uses ‘whisper,’ which has a wide range of support for low-resource languages. However, the ASR performance for low-resource languages (Pakistani Mardu, Hindi, Moroccan Arabic et.al) is not as strong as it is for widely spoken languages like English, which have more data available. In specific scenarios involving low-resource languages, domain-specific adaptation can be applied using data from those languages.
Domain adaptation for ASR (Automatic Speech Recognition) of low-resource languages refers to the process of adapting automatic speech recognition technology to low-resource or specific domains. Low-resource languages (Pakistani Mardu, Hindi, Moroccan Arabic et.al) typically refer to those with fewer speakers compared to major world languages like English, Chinese, and Spanish. Due to the limited data resources for these languages, directly training efficient ASR models can be challenging.
The purpose of domain adaptation here is to utilize a large number of existing resources (like pre-trained models for major languages) to enhance the ASR performance for low-resource languages or specific domains. Here are some commonly used strategies:
• Transfer Learning: First pre-train an ASR model on a major language, then fine-tune it using data from the low-resource language.
• Multi-task Learning: Train the model to handle tasks in multiple languages simultaneously, allowing it to learn common features from various languages.
• Data Augmentation: Increase the training data volume for low-resource languages using techniques like speed change, pitch alteration, adding noise, etc.
• Simulated Data: Use Text-to-Speech (TTS) technology to generate simulated voice data for
low-resource languages.
• Adaptive Front-end: Design an acoustic model frontend that can adapt to different language characteristics.
• Multi-model Fusion: Combine the outputs of multiple models to improve recognition accuracy.
• Semi-supervised Learning: Train the model using a large amount of unlabeled data from low-resource languages combined with a small amount of labeled data.
Regardless of which strategy is adopted, a small but accurately annotated dataset of the low-resource language is indispensable to adapt the pre-trained large models. This emphasizes the importance of precise annotation and collection of data for these languages. Such data is invaluable in the field of voice recognition because it provides the necessary “guidance” for the model to better understand and process low-resource languages.
DataOceanAI has nearly 20 years of accumulation in the field of AI training data, covering more than 200 global languages and dialects, and has accumulated nearly 1500 off-the-shelf datasets with 100% exclusively-owned IP rights, we also continue to invest in research and development and launches small-language datasets every year. These datasets provide valuable resources for research institutions and support the industry in transferring large models to applications in low-resource language scenarios.
Bosnian Speech Recognition Corpus – King-ASR-823 >>>Learn More
This is a 1-channel Bosnian mobile phone speech database, collected in a quiet office/home environment. The corpus contains about 100 hours and is composed of 177 speakers. The corpus domain involves News, Travel, Economy, Entertainment, Sport, Technology, Conversation, Independent Words. The entire database includes dataset related files such as recordings, scripts, pronunciation lexicon, and speaker information.
Hindi Speech Recognition Corpus – King-ASR-817 >>>Learn More
This is a 1-channel Indian Hindi mobile phone speech database, which is collected in quiet office environment. The corpus contains about 1225 hours, which were from 721 speakers. The domain of corpus involves Daily Conversation, Shopping and tourism, Numbers and Times, Medical and Covid 19, Education and Study, Name and Locations, Political and Diplomacy, Technology and Games, Sports and Entertainment, Society and Economy. The entire database includes dataset related files such as recordings, scripts, pronunciation lexicon, and speaker information.
Learn more ASR corpus:ASR – DataoceanAI