With the continuous advancement of AI large language models, the significance of data is becoming increasingly apparent. Recently, the concept of “data-centric AI” has been frequently mentioned in academic papers.
Data-centric AI refers to the framework for developing, iterating, and maintaining data for AI systems. Data-centric AI involves tasks and methods related to constructing effective training data, designing appropriate inference data, and maintaining data. Data-centric AI places a strong emphasis on researching how to efficiently build high-quality and large-scale datasets.
This data-centric approach does not diminish the value of model-centric AI; instead, these two paradigms intertwine and complement each other when building AI systems.
On one hand, we can employ model-centric approaches to achieve data-centric AI goals. For instance, we can use generative models like GANs and diffusion models to perform data augmentation and generate more high-quality data.
On the other hand, data-centric AI can drive improvements in model-centric AI objectives. For example, enhancing data availability may stimulate further advancements in model design. Consequently, in real-world applications, data and models often evolve in a mutually reinforcing and evolving environment.
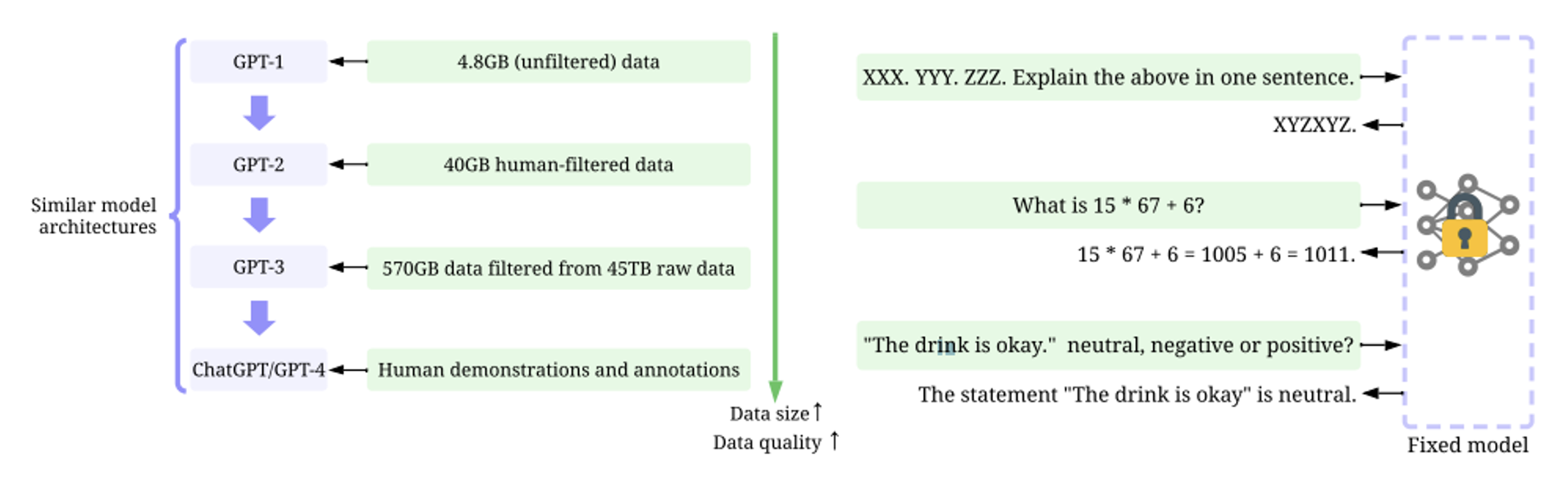
From: Data-centric AI: Survey
The importance of data is increasingly evident, especially with the development of large language models in recent years, as depicted in the iterative process of ChatGPT shown in the above image. The data required for training has not only improved in quality but has also witnessed explosive growth in quantity.
In addition to data used for training, inference data has also become a continuously evolving domain, such as in the field of engineering for ChatGPT. As the value of data becomes more pronounced, many research departments are embarking on foundational work, including methods for data collection and the creation of debugging data.
01 Data-centric AI
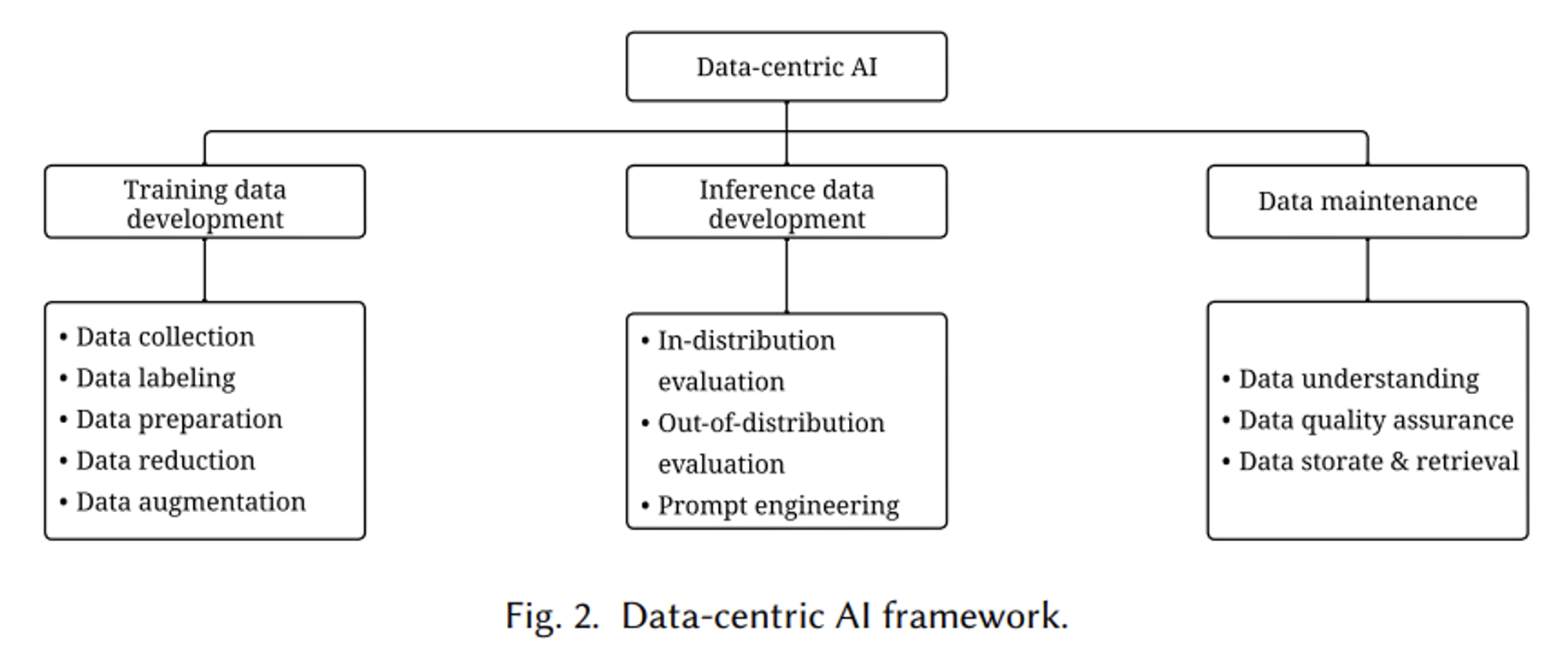
From: Data-centric AI: Survey
Data-centric AI comprises three aspects: training data development, inference data development, and data maintenance.
The goal of training data development is to collect and produce rich, high-quality training data to support AI model training. It consists of five sub-goals, including data collection, data labeling for information tagging, data preparation for cleaning and transformation, further processing of raw data, and enhancing data diversity without the need for additional data collection.
The objective of inference data development is to create a reasonable evaluation dataset for providing a rational assessment and testing of the model. This includes three goals: testing with a test set from the same distribution, testing with test sets from different distributions, and engineering testing.
Data maintenance refers to the continuous updating and maintenance of data. In practical applications, data is not a one-time creation but requires ongoing maintenance. The purpose of data maintenance is to ensure the quality and reliability of data in dynamic environments. It involves three fundamental sub-goals: data understanding, which aims to provide visualization and assessment of complex data for valuable insights; the formulation of quantitative measurement and quality improvement strategies to monitor and rectify data while ensuring data quality; and the design of data storage and retrieval methods, with the goal of designing efficient algorithms to provide the required data by appropriately allocating resources and efficiently handling queries. Data maintenance plays a foundational and supporting role in the data-centric AI framework, ensuring the accuracy and reliability of data in both training and inference.
02 Training Data Development Process
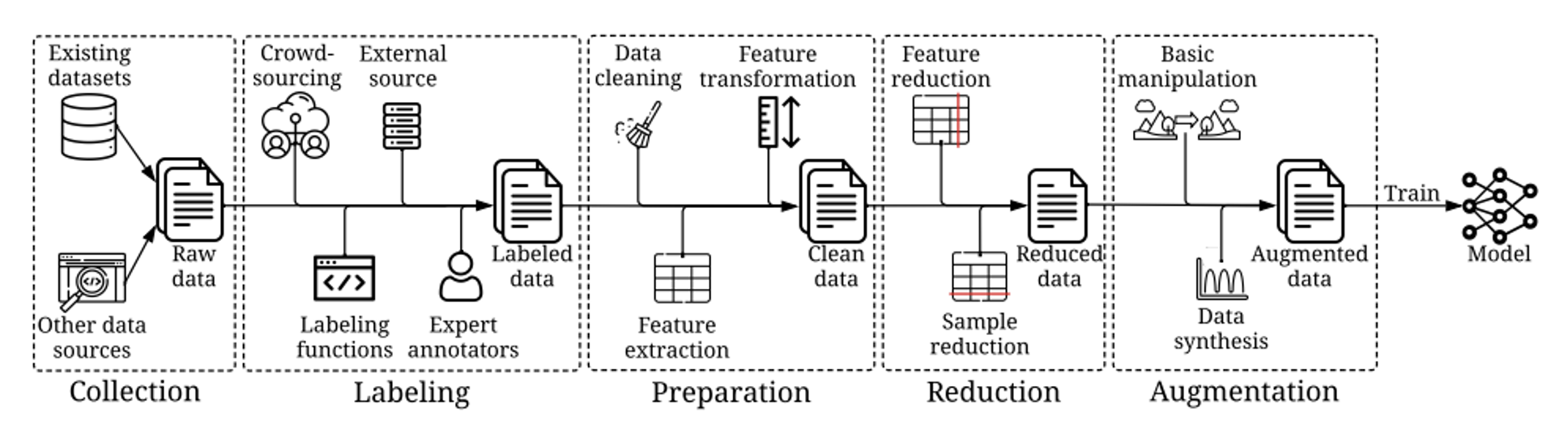
From: Data-centric AI: Survey
The diagram above illustrates the training data development process, which includes data collection, labeling, preparation, refinement, and augmentation.
Data collection refers to the process of gathering data from various sources, and this step fundamentally determines the quality and quantity of the data. This stage often requires a strong domain expertise, as a deep understanding of the domain is necessary to prepare the data in a way that aligns with the desired outcomes. It typically involves data gathering, data normalization, and synthetic data creation.
Data labeling involves attaching labels to the data, with these labels being directly used for training models. While some unsupervised learning has achieved significant success, achieving results that align well with human performance often requires supervised learning with labeled data.
The data preprocessing process mainly includes data cleaning, feature extraction, and feature transformation.
Data refinement refers to data dimensionality reduction, with the primary goal of reducing the complexity of a given dataset while retaining its essential information. This can typically be achieved by reducing feature or sample size.
Data augmentation aims to improve model performance by increasing data samples and diversity. It’s important to note the distinction between data augmentation and data reduction; while they may seem opposed, their focus is different. Data reduction primarily aims to eliminate information redundancy, whereas data augmentation focuses on increasing information content.
03 Automation or Collaboration
Data collection comes in two forms: automated processing and human involvement. Currently, the training data for large language models relies mostly on automated scripts for crawling and labeling.
Automated Approaches
• Programmatic automation: Data is automatically processed using programs, often designed based on heuristics and statistical information.
• Learning-based automation: Automation strategies are learned through optimization, such as minimizing a specific objective function. These methods are typically more flexible and adaptive but require additional learning costs.
• Pipeline automation: A series of strategies are integrated and adjusted across multiple tasks, helping to determine the globally optimal strategy.
Human Involvement Approaches
• Full human involvement: Human participation is required at every step, aligning well with human intent but incurring higher costs.
• Partial human involvement: Humans provide information intensively or continuously, often through extensive feedback or frequent interactions.
• Minimal human involvement: Humans are consulted only when needed. They participate when ed or requested, suitable for scenarios with abundant data and limited human resources.
While data-centric AI is relatively new, significant progress has already been made in various related tasks. In most cases, this progress is viewed as a preprocessing step within the model-centric paradigm. Data-centric AI not only demands large amounts of data but also requires high data quality, often necessitating the involvement of professional data companies to ensure data quality.
DataOceanAI is a company dedicated to improving AI data. It provides a wide range of precision-annotated databases for AI model training, including ASR, TTS, SV, SD, CV, Text, and various multi-modal datasets and data services.
References
Zha, Daochen, Zaid Pervaiz Bhat, Kwei-Herng Lai, Fan Yang, Zhimeng Jiang, Shaochen Zhong, and Xia Hu. “Data-centric artificial intelligence: A survey.” arXiv preprint arXiv:2303.10158 (2023).