Intelligent speech transcription refers to the process of using artificial intelligence technology to convert voice signals into text information. It involves technologies in various fields such as speech recognition, language understanding, and natural language processing.
Intelligent speech transcription not only achieve high-precision speech recognition but also make intelligent judgments and processing based on context, improving the accuracy and efficiency of voice-to-text conversion. With the development of artificial intelligence and the implementation of LLM, the accuracy and robustness of intelligent speech transcription are becoming increasingly better.
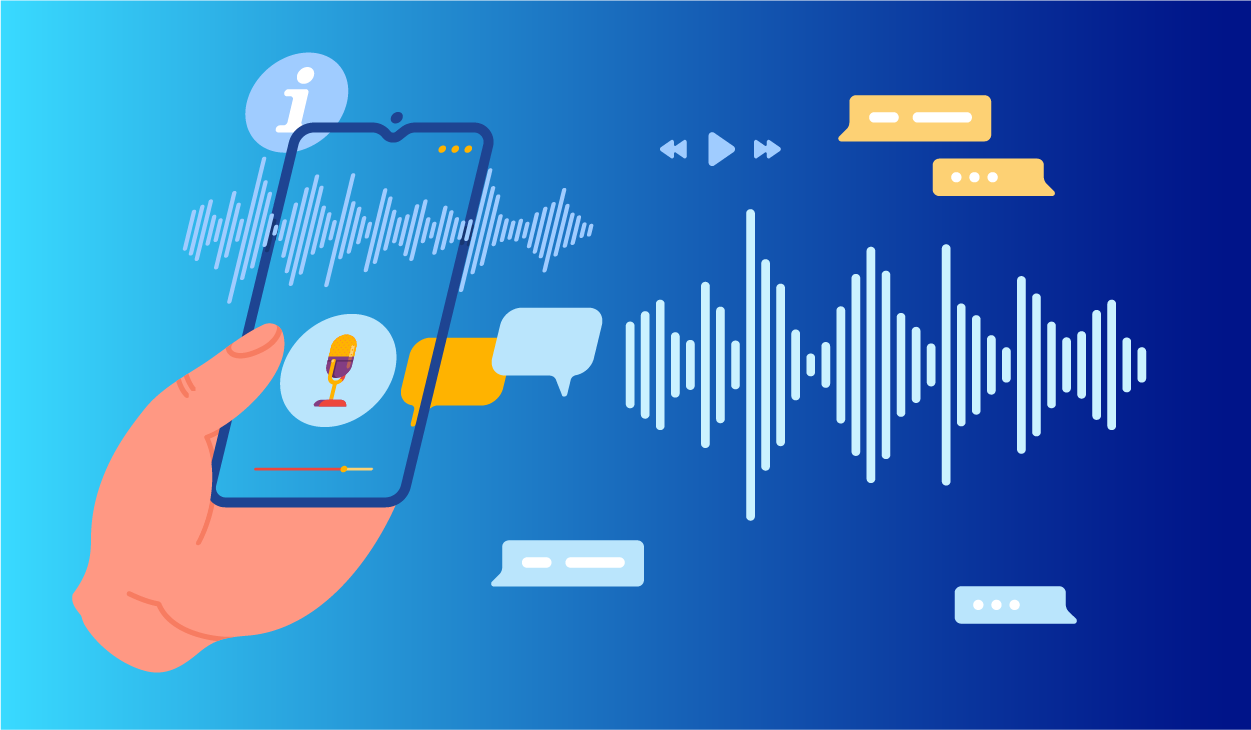
How LLM Assist Intelligent Speech Transcription
With the rapid development of AI and the emergence of various LLM, the accuracy and robustness of intelligent speech transcription have been improved and progressed with the addition of LLM. Models like Whisper and ChatGPT, trained on extensive data, can provide higher accuracy in speech recognition and transcription services. These models excel in understanding different accents, dialects, and speech contexts. Particularly, models like ChatGPT demonstrate remarkable performance in contextual understanding, contributing to improved accuracy and relevance in speech transcription. These models typically support multiple languages, making speech transcription services accessible to a wider user base. Their natural language processing capabilities aid in handling grammar and semantics more effectively, resulting in smoother and more natural text output during transcription.
Challenges in Implementing LLM for Intelligent Speech Transcription
While models like Whisper and ChatGPT significantly assist in intelligent speech transcription, they also pose new challenges:
• Multi-Speaker Environments
Distinguishing and accurately transcribing speech in environments with multiple speakers or significant background noise remains a challenge. Overlapping speech and interference in such environments may lead to transcription errors.
• Dialects and Accents
Despite the adaptability of LLM to multiple languages and accents, they may struggle with region-specific dialects or heavy accents. Slang, informal expressions, or fragmented sentences in everyday conversation may be challenging for the model to accurately understand and transcribe.
• High Computational Costs
LLM require substantial computational resources for speech processing and analysis, potentially leading to high operational costs, especially in real-time transcription applications. The increased demand for computational resources may impact environmental factors, particularly in terms of energy consumption and carbon emissions in data centers. This can be a limiting factor for small businesses or individual users, especially in scenarios requiring on-premise servers for speech processing.
• Accuracy of Specialized Terminology
In professional fields such as medicine and law, accurate recognition and transcription of specialized terminology are crucial. LLM may need specific training and optimization for these domains. Different industries have unique contexts and expressions, requiring models to understand and adapt to these specific contexts for accurate transcription. Continuous learning and updates are necessary to keep up with industry changes and new expressions.
Strategies to Address Challenges
• Multi-Speaker Environments
Collect data that includes speech from multiple speakers, especially in noisy backgrounds, to train models better in distinguishing and handling different speakers. Integrate speaker recognition technology to differentiate speakers and improve transcription accuracy.
• Dialects and Accents
Collect data specific to dialects and accents that LLM may struggle with. Customize model training with additional samples to enhance its ability to understand and process region-specific dialects and accents. Include training data with slang, colloquial expressions, and other informal language to improve the model’s understanding and processing capabilities.
• Accuracy of Specialized Terminology
Collect domain-specific data, especially in fields like medicine and law, containing relevant terminologies to train the model. Collaborate with industry experts to ensure the accuracy and professionalism of the transcription. Purchase customized datasets tailored to specific industries, including industry-specific expressions and contexts.
It’s crucial to note that acquiring specialized data requires collaboration with professional companies for efficient and cost-effective data collection. DataOceanAI as an experienced data company, offers a wide range of speech recognition datasets covering various scenarios, languages, and dialects. Their datasets are diverse, accurately annotated, and contribute to enhancing model robustness and domain-specific applications. Meanwhile, we provide professional speech transcription services to satisfy the needs of customers in different industries.