Automatic Speech Recognition (ASR) for meeting scenarios is an advanced voice processing technology designed for multi-party conference environments. Its core goal is to solve the problem of ‘who said what and when,’ meaning it accurately identifies and records the speech of each participant in a meeting, and marks the identity and speaking time of each speaker.
ASR for meetings is a classic cocktail party problem. This technology has a wide range of applications, including automatic recording and transcription of meeting content, real-time subtitle generation, multilingual translation, emotion analysis, support for information retrieval and knowledge management, improvements in education and training, and in legal and judicial fields for recording statements and debates.
The current popular ASR systems for meeting scenarios typically adopt a multi-module coupled architecture, which includes front-end voice processing, speaker separation, speaker identification and labeling, speech recognition, and post-processing. It is challenging to achieve end-to-end optimization among these modules.
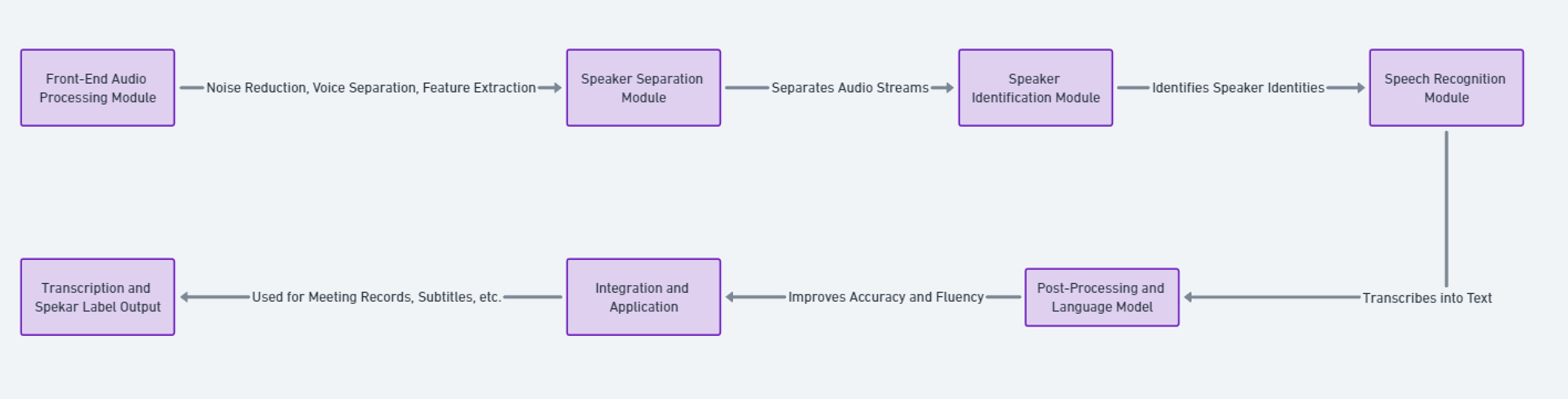
01 The Challenges of ASR in Meeting Scenarios
Due to the complex multi-person, multi-scene, multi-device, and high real-time requirements of the conference scene, there are many challenges faced by the Automatic Speech Recognition (ASR) system:
• Multi-speaker recognition: In meetings, multiple people speak at the same time, and the ASR system needs to accurately distinguish the voices of different speakers and associate them with the correct identities. This involves modeling the voice characteristics of speakers and confirming their identities.
• Overlapping speech processing: It is common for multiple people to speak at the same time in meetings, leading to overlapping speech signals. The ASR system needs speech separation technology to divide overlapping speech into separate signals for recognition and transcription.
• Unknown number of speakers: The number of participants in a meeting is often uncertain, and the ASR system needs to dynamically adapt to different numbers of speakers without losing accuracy.
• Far-field pickup: In conference rooms, microphones are often far from the speakers, resulting in decreased voice signal quality. The ASR system requires far-field voice pickup technology to improve signal quality and enhance recognition accuracy.
• Noise and reverberation: Various noises and reverberations, such as background noise and echoes, may be present in conference rooms. The ASR system needs to resist these interferences to maintain voice recognition performance.
• Algorithmic challenges: The coupling of multiple modules prevents the model from achieving end-to-end optimization, and it is impossible to obtain the globally optimal result for the entire system.
•Project landing challenges: There is a lack of real recording data for conference scenarios, hindering scientific research exploration and practical implementation.
02 The Potential Unleashed by Advanced LLM
Recently, the field of speech recognition has entered an era dominated by LLMs such as Whisper, wavLM, and wav2vec. These advanced speech models are significantly improving recognition accuracy and effectiveness, especially in meeting scenarios.
Whisper, in particular, underwent pre-training with 680,000 hours of diverse voice data across 98 languages, numerous dialects, and various settings. It exhibits robust multi-scenario and multi-task recognition capabilities and powerful discriminative representation skills.
Whisper is specifically optimized for multilingual automatic speech recognition (ASR), speech translation (ST), and language identification (LI). Large speech models like Whisper achieve higher accuracy in voice recognition due to their ability to learn more language knowledge and contextual information. This enhances the performance of ASR modules, enabling more accurate voice content recognition in multi-speaker environments. Numerous studies have adapted Whisper for speaker detection tasks, demonstrating its ability in speaker recognition, which aids in speaker labeling tasks.
For example, a recent paper introduced a lightweight adapter module to migrate Whisper to multi-speaker scenarios. This approach maintains the model’s multilingual properties even when adapted using a single language. The study also developed an enhanced serialized output training for performing multi-speaker ASR and simultaneous speech timestamp prediction. This involves predicting all speakers’ ASR hypotheses, calculating the number of speakers, and estimating speech timestamps simultaneously.
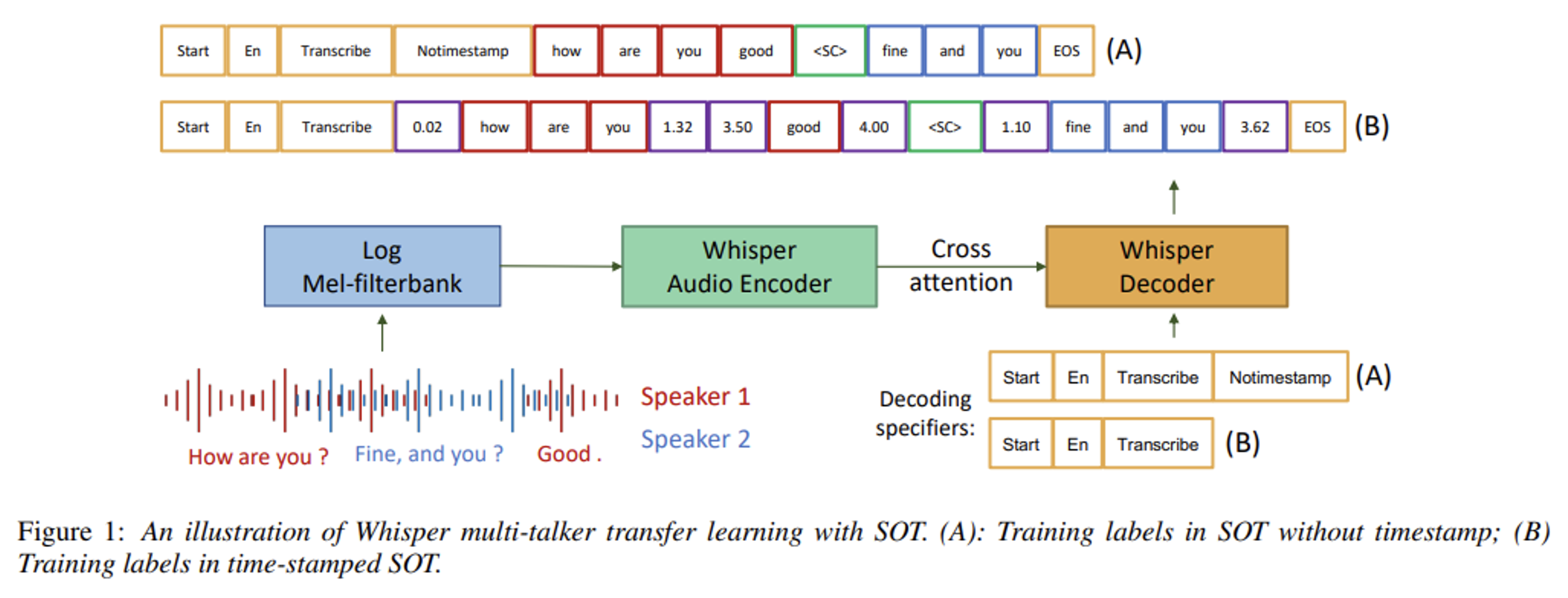
From “Adapting Multi-Lingual ASR Models for Handling Multiple Talkers”
Despite the potential benefits large speech models offer for voice recognition in meeting scenarios, it’s important to note that these models may require in-domain data from meeting contexts for migration and adaptation, to make Whisper compatible with multi-speaker ASR applications and acoustic environments. Therefore, actual recorded data from various scenarios and languages in meeting contexts is essential.
03 Release of ASR Data for Conference Scenario
Despite large speech models like Whisper showing great potential in speech recognition for conference scenes, we must recognize that to achieve optimal performance in multi-speaker ASR applications, these models often require transfer learning and adaptive adjustments specifically for certain conference scenarios.
The key to this process lies in having a rich variety of actual recording data from conference scenes, especially data covering a range of languages and acoustic environments. The diversity and authenticity of these data are crucial for the effective training of models, as they provide the real-world conditions that the models need to learn and adapt to.
Without the support of these specialized datasets, even the most advanced models may fail to accurately recognize and process the multiple voice overlaps in complex conference scenarios. Therefore, collecting and utilizing high-quality conference scene ASR data is not only key to enhancing model performance but also an important step in advancing speech recognition technology.
Based on the above discussion on the importance of conference scene ASR data, DataOceanAI has launched the following datasets, which are particularly crucial for developing and optimizing multi-language, multi-speaker Automatic Speech Recognition (ASR) systems:
King-ASR-835: Japanese Business Meeting Conversational Speech Recognition Corpus
This dataset focuses on American English business meeting dialogues, also recorded using mobile phones. It provides essential data for ASR systems to understand and process English meeting dialogues, especially in situations involving different accents and speaking styles.
King-ASR-867: American English Business Meeting Conversational Speech Recognition Corpus
This dataset contains Mandarin Chinese business meeting dialogues collected using various recording devices such as headset mics, recording pens, and pickups. The diversified recording methods help ASR systems better adapt to different acoustic environments, improving accuracy and reliability in practical applications.
King-ASR-856: Chinese Mandarin Business Meeting Conversational Speech Recognition Corpus
This dataset provides recordings of business meeting dialogues in Japanese, particularly using mobile phones, covering three-person group exchanges. This type of dataset is valuable for training ASR systems to recognize and process speech interactions in Japanese meetings, especially in simulated real meeting environments.
The diversity of these datasets and their focus on specific languages and scenarios make them valuable resources for developing efficient, accurate multi-language conference scene ASR systems. By utilizing these datasets for in-depth training and testing, ASR systems’ performance in handling multi-speaker, multi-language meeting environments can be significantly enhanced, thus advancing the field of speech recognition.”